In the world of Artificial Intelligence (AI), two buzzwords have been making waves—Generative AI and Predictive AI. From personalized shopping suggestions to AI-generated art, these technologies are transforming industries and redefining what's possible with data. But as these terms are often used interchangeably, many are left wondering: What is the difference between generative AI and predictive AI?
Understanding the distinction between generative AI vs predictive AI is not just a matter of tech jargon—it’s the key to unlocking the true potential of AI in solving real-world problems. Whether you're a developer, data scientist, business leader, or simply AI-curious, knowing when to use each type of AI can make all the difference in achieving your goals.
In this comprehensive guide, we’ll explore:
- What each type of AI is
- How they work under the hood
- Where they're applied in real life
- And most importantly, what is the difference between generative and predictive AI
Let’s dive into the exciting world of AI—where machines don’t just think, they predict, and sometimes, they even create.
Also Read: What is Gradient Descent in Deep Learning? A Beginner-Friendly Guide
What is Predictive AI?
Predictive AI is a branch of artificial intelligence that focuses on forecasting future outcomes based on historical and current data. Think of it as a smart system that looks at patterns in the past and says, “Here’s what’s likely to happen next.”
At its core, Predictive AI doesn’t create new content—it makes intelligent guesses about the future. It’s widely used in industries like finance, healthcare, marketing, and e-commerce, where decisions rely heavily on data-driven predictions.
How Predictive AI Works
Predictive AI uses machine learning algorithms that are trained on labeled datasets. These models identify trends, correlations, and probabilities from past data to estimate what’s coming next.
Here’s a simplified breakdown:
- Data Collection: Historical data is gathered (e.g., past sales records, weather data, or user behavior).
- Training the Model: A predictive model learns the relationships between input features and outcomes.
- Prediction: When new data is introduced, the model applies learned patterns to predict an outcome.
Common Predictive Algorithms
- Linear Regression – For predicting numerical values (e.g., house prices).
- Logistic Regression – For binary classification (e.g., spam or not).
- Decision Trees and Random Forests – For complex predictions with multiple variables.
- Time Series Models (ARIMA, Prophet) – For forecasting trends over time.
- Neural Networks – For learning non-linear, deep patterns in data.
Real-World Applications of Predictive AI
- Retail: Forecasting customer demand and inventory needs.
- Healthcare: Predicting disease outbreaks or patient readmissions.
- Finance: Credit scoring and stock price prediction.
- Marketing: Anticipating customer churn or campaign performance.
- Manufacturing: Predictive maintenance of machinery.
Also Read: Everything You Need To Know About Optimizers in Deep Learning
Predictive AI is all about insights and foresight—it helps businesses act proactively rather than reactively. But unlike its creative cousin, generative AI, it doesn’t make something new. It informs, guides, and supports strategic decision-making.

What is Generative AI?
While Predictive AI focuses on forecasting, Generative AI takes a creative leap—it’s designed to generate entirely new content based on patterns learned from existing data. Instead of just telling you what might happen next, it creates something that has never existed before, like a new image, poem, song, or even computer code.
In simple terms, Generative AI answers the question:
“Given what I’ve learned, what can I create?”
How Generative AI Works
Generative AI models are trained on large datasets such as text documents, images, or audio files. These models learn the structure, style, and nuances of the data, enabling them to synthesize new outputs that resemble the training data but are not copied from it.
Here’s how it works:
- Training on Large Data: The model ingests vast amounts of unstructured data (like books, websites, or images).
- Learning Patterns: It understands language, tone, colors, shapes, or sequences depending on the data type.
- Generating Content: Given a prompt or input, it produces new text, visuals, or sounds that feel original and context-aware.
Popular Generative AI Models & Techniques
- Transformer Models (e.g., GPT-4, BERT, T5): For generating human-like text
- GANs (Generative Adversarial Networks): For creating images and videos
- VAEs (Variational Autoencoders): For encoding and generating variations of input data
- Diffusion Models (e.g., Stable Diffusion, DALL·E): For detailed and high-quality image generation
Real-World Applications of Generative AI
- Content Creation: Writing blogs, marketing copy, and scripts
- Image & Art Generation: Creating realistic or artistic images (Midjourney, DALL·E)
- Music Composition: Generating melodies or full tracks
- Code Assistance: Autocompleting or generating code (GitHub Copilot)
- Gaming & Design: Creating characters, levels, and visual assets
- Chatbots: Powering human-like conversations (ChatGPT, Bard)
Generative AI is the creative engine of the AI universe. It brings imagination and automation together, opening doors for writers, designers, developers, and anyone looking to build or express something new.
Generative AI vs Predictive AI: Key Differences
Now that we’ve explored both worlds, let’s break down the differences between generative AI and predictive AI. While they may seem similar at first glance—they both learn from data—their goals, outputs, and applications are entirely different.
Here's a side-by-side comparison to make it crystal clear:
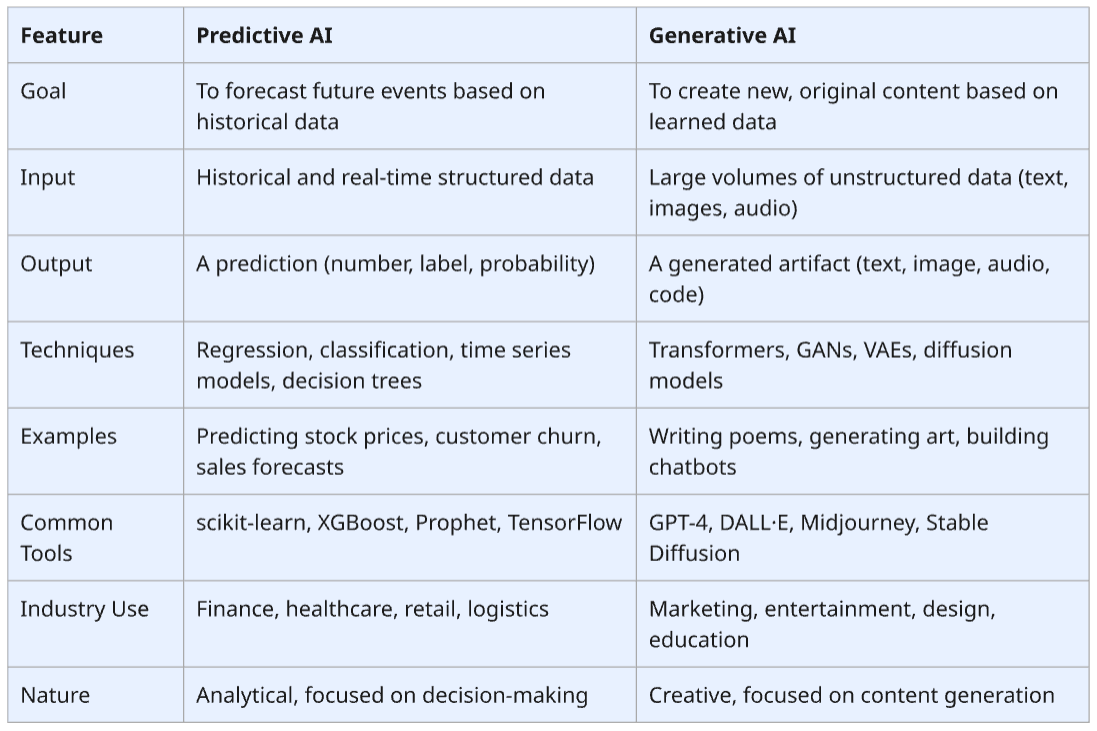
- Predictive AI is like a smart analyst—it helps you make better decisions based on what it sees in the past.
- Generative AI is like a creative artist—it produces brand-new content that never existed before.
Understanding generative AI and predictive AI helps businesses, developers, and creatives choose the right tool for the right task.
Also Read: How Computer Vision Is Transforming Industries: Examples from Healthcare to Retail
When to Use Generative AI vs Predictive AI
Choosing between generative AI and predictive AI depends on your goal, the type of output you need, and the kind of data you have. While both are powerful, they shine in different problem spaces.
Here’s how to know which one to use—and when.
Use Predictive AI When You Need:
- Forecasting or Classification
- Example: Predicting next month’s revenue, identifying fraudulent transactions.
- Example: Predicting next month’s revenue, identifying fraudulent transactions.
- Decision Support
- Example: Recommending products to customers based on purchase history.
- Example: Recommending products to customers based on purchase history.
- Risk Assessment
- Example: Predicting loan defaults, disease risk scores, customer churn.
- Example: Predicting loan defaults, disease risk scores, customer churn.
- Optimization and Planning
- Example: Route optimization for deliveries or resource allocation.
- Example: Route optimization for deliveries or resource allocation.
Perfect for: Analysts, operations teams, financial forecasters, and data scientists who need accurate and explainable insights.
Use Generative AI When You Need:
- Content Creation
- Example: Writing blogs, composing music, creating visual designs.
- Example: Writing blogs, composing music, creating visual designs.
- Human-Like Interaction
- Example: Building intelligent chatbots or virtual assistants.
- Example: Building intelligent chatbots or virtual assistants.
- Prototyping & Design
- Example: Generating mockups, user interfaces, or ad creatives.
- Example: Generating mockups, user interfaces, or ad creatives.
- Data Augmentation
- Example: Generating synthetic data to improve machine learning models.
- Example: Generating synthetic data to improve machine learning models.
Perfect for: Creators, marketers, developers, product designers, and innovators looking to automate and scale creativity.
Choosing the Right AI
Ask yourself:
- Do I want to predict something? → Use Predictive AI
- Do I want to create something new? → Use Generative AI
Sometimes, the two can work together. For example, a predictive model might identify trending topics, while a generative model writes an article about them.
Real-World Examples: Generative AI vs Predictive AI in Action
To truly understand generative AI vs predictive AI, let’s dive into some real-world scenarios where these technologies are making a huge impact.
Predictive AI in Retail: Forecasting Demand
Example: In retail, predictive AI helps businesses forecast product demand and manage inventory efficiently. For instance, an e-commerce company can use historical sales data to predict which products will be in demand during upcoming seasons. This allows them to adjust stock levels and avoid overstocking or stockouts, ensuring better operational efficiency.
- How it works: The AI model analyzes past sales patterns, seasonality, and external factors like promotions, weather, or market trends to predict future demand.
- Real-World Tool: Tools like Prophet and TensorFlow are used to train predictive models in retail businesses.
Generative AI in Content Creation: Automated Writing
Example: One of the most popular applications of generative AI is in automated content generation. Platforms like GPT-3 (and its successors) allow businesses to create blog posts, social media content, and marketing copy automatically, based on a few input parameters.
- How it works: Generative models trained on large text corpora understand language structure and can generate human-like, coherent content.
- Real-World Tool: Tools like Copy.ai and Jasper use GPT-3 and other generative models to craft compelling copy.
Predictive AI in Healthcare: Disease Prediction
Example: In healthcare, predictive AI is being used to forecast the likelihood of diseases or conditions based on a patient’s medical history and other data points. For instance, predictive models can assess the risk of heart disease by analyzing patient records, lifestyle factors, and genetic predispositions.
- How it works: By analyzing historical health data, predictive models identify patterns that help doctors predict future health issues, enabling early intervention.
- Real-World Tool: IBM Watson Health and Google Health use predictive AI for early diagnosis and treatment recommendations.
Generative AI in Art & Design: AI-Generated Artwork
Example: Generative AI has found a powerful niche in the creative industries, especially in art and design. DALL·E, a generative AI model, can create stunning visuals based on simple text prompts. For instance, users can ask for “a futuristic cityscape,” and DALL·E will generate a detailed image of what that might look like.
- How it works: Using deep learning, generative models like GANs (Generative Adversarial Networks) learn the intricacies of art, producing new images that mimic real-world designs, yet remain entirely original.
- Real-World Tool: Midjourney, DeepArt, and Artbreeder offer creative professionals AI-generated visuals for projects and inspiration.
Predictive AI in Autonomous Vehicles: Predicting Traffic and Hazards
Example: Self-driving cars use predictive AI to navigate and avoid hazards on the road. Predictive models analyze historical traffic patterns, weather data, and current road conditions to make real-time decisions.
- How it works: By collecting real-time sensor data, predictive models identify the safest route, anticipate potential accidents, and adjust speed for optimal driving.
- Real-World Tool: Companies like Tesla and Waymo use predictive models to drive autonomous vehicles safely.
Also Read: What is LLM? A Complete Guide to Large Language Models in AI and Generative AI
Generative AI in Chatbots: Conversational AI
Example: Generative AI powers conversational agents (chatbots) that mimic human-like interaction, providing support in industries like customer service, healthcare, and retail. ChatGPT is a prime example of this, where the AI generates natural responses to users’ queries.
- How it works: The model uses its knowledge of language to generate appropriate, contextually aware responses in real-time.
- Real-World Tool: ChatGPT, Dialogflow, and Rasa are widely used in building intelligent virtual assistants.
Conclusion
In this article, we explored what is the difference between generative AI and predictive AI and how these two types of artificial intelligence serve distinct but complementary purposes.
While they serve different purposes, predictive AI and generative AI can often work in tandem. For example, a predictive AI model might predict a trend, while generative AI can be used to create content based on that prediction.
Both technologies are transforming industries, enabling businesses and individuals to enhance their capabilities and create innovative solutions that were once beyond imagination. As AI continues to evolve, the line between the two will blur, leading to even more advanced applications.
Ready to transform your AI career? Join our expert-led courses at SkillCamper today and start your journey to success. Sign up now to gain in-demand skills from industry professionals. If you're a beginner, take the first step toward mastering Python! Check out this Full Stack Computer Vision Career Path- Beginner to get started with the basics and advance to complex topics at your own pace.
To stay updated with latest trends and technologies, to prepare specifically for interviews, make sure to read our detailed blogs:
How to Become a Data Analyst: A Step-by-Step Guide
How Business Intelligence Can Transform Your Business Operations