In the realm of deep learning, data is everything. The more diverse and comprehensive your dataset, the better your model performs. But what happens when you don’t have access to massive datasets? Or when collecting new data is expensive, time-consuming, or even impossible?
This is where data augmentation in deep learning steps in as a game-changer.
What is data augmentation in deep learning? Simply put, it's a technique that allows you to artificially increase the size and variety of your training data by applying transformations like rotation, flipping, noise addition, and more — all without collecting new data. These techniques not only help your model learn better but also improve its ability to generalize to unseen scenarios.
In this article, we'll explore the importance of data augmentation, look at the most popular data augmentation techniques in deep learning, and understand how they’re applied across different domains like computer vision, natural language processing, and audio analysis — all explained with clear examples.
Also Read: What is Gradient Descent in Deep Learning? A Beginner-Friendly Guide
Why Is Data Augmentation Important in Deep Learning?
Deep learning models are known for their ability to learn complex patterns — but they also have a downside: they can easily overfit if the training data is limited or lacks diversity. Overfitting happens when a model learns the noise or irrelevant details in the training data instead of the actual patterns, which leads to poor performance on new, unseen data.
This is where data augmentation becomes essential.
Here’s why data augmentation is so important:
1. Reduces Overfitting
By introducing variations in the training data (like rotating or flipping an image), the model is exposed to a wider range of inputs, making it less likely to memorize and more likely to generalize.
2. Handles Limited Datasets
In many real-world scenarios — like medical imaging or rare language dialects — collecting large datasets is not feasible. Data augmentation helps you make the most of what you have by generating more diverse samples from the existing data.
3. Improves Model Robustness
Augmented data prepares the model for real-world situations by simulating possible variations it might encounter, such as noise, different lighting conditions, or typos in text.
4. Balances Imbalanced Classes
If your dataset has class imbalance (e.g., 90% cats and only 10% dogs), data augmentation can be applied more to the minority class to help the model learn fairly.
In short, data augmentation isn’t just a bonus — it’s often a necessity to train deep learning models that are accurate, reliable, and ready for real-world deployment.
How Does Data Augmentation Work?
The core idea of data augmentation is simple yet powerful: apply various transformations to your existing training data in ways that preserve the original label but introduce diversity in form.
Imagine you have a dataset of 1,000 cat images. By rotating, flipping, zooming in, or changing the brightness of these images, you could easily generate 5,000 or more unique images — all still labeled “cat.” That’s the magic of augmentation.
Let’s break it down by data type:
For Image Data
You can apply transformations such as:
- Rotating the image slightly
- Flipping it horizontally or vertically
- Adjusting brightness or contrast
- Adding small amounts of random noise
Also Read: Everything You Need To Know About Optimizers in Deep Learning
A photo of a dog rotated 15 degrees is still a dog. The label doesn't change, but the variation makes the model stronger.
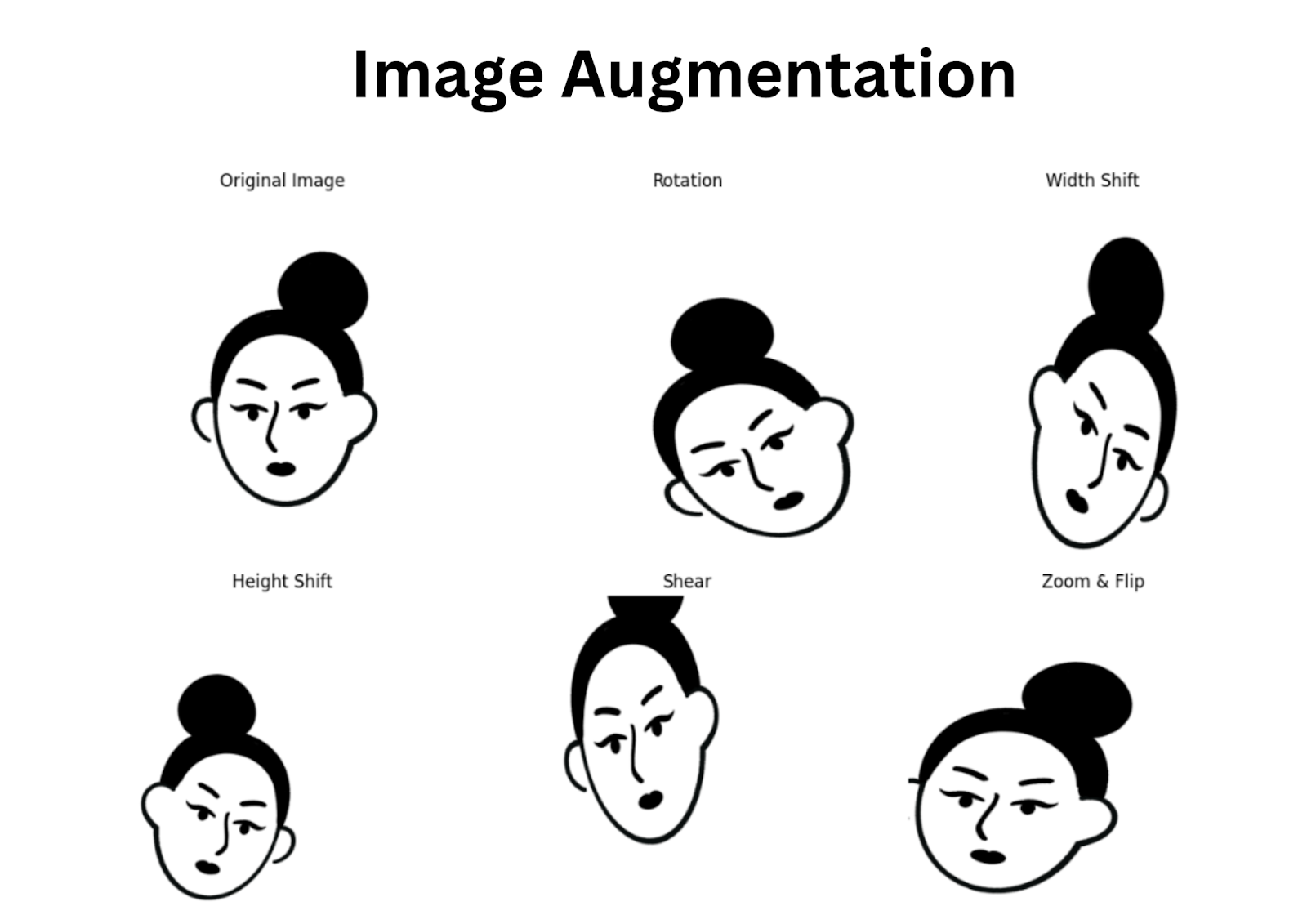
For Text Data
Text augmentation is trickier but highly effective. Some common methods include:
- Synonym Replacement: “Happy” becomes “joyful.”
- Random Insertion/Deletion: Adds or removes words without changing meaning.
- Back Translation: Translate a sentence to another language and back to rephrase it.
Example: “I love this product” → “This product is amazing.”
For Audio Data
In speech and audio processing, augmentation can mimic different environments by:
- Adding background noise
- Stretching or compressing time
- Shifting pitch
A voice clip recorded in a quiet room vs. a noisy cafe — both can be used to train a robust voice assistant.
Each of these techniques allows the model to experience a broader range of input variations, helping it generalize better when faced with new data during real-world use.
Also Read: How Computer Vision Is Transforming Industries: Examples from Healthcare to Retail
Popular Data Augmentation Techniques in Deep Learning
Now that you understand how data augmentation works, let’s dive into the most commonly used data augmentation techniques, categorized by data type: image, text, and audio.
Image Data Augmentation Techniques
Image augmentation is the most widely used and well-supported in deep learning libraries like TensorFlow, Keras, and PyTorch. Some popular techniques include:
Rotation
Rotating an image by a few degrees to simulate different viewing angles.
Flipping
Horizontal and vertical flips help models understand symmetry — for example, a cat facing left or right is still a cat.
Cropping and Zooming
Random cropping helps focus on different parts of the object, while zooming simulates distance variation.
Color Jittering
Changes in brightness, contrast, saturation, or hue mimic real-world lighting conditions.
Noise Injection
Adding Gaussian or salt-and-pepper noise makes the model resistant to real-world imperfections like camera grain.
These techniques are essential in computer vision tasks such as object detection, classification, and segmentation.
Text Data Augmentation Techniques
Text data is more delicate since minor changes can alter the meaning. Still, powerful methods exist:
Synonym Replacement
Randomly replaces words with their synonyms to retain meaning while diversifying vocabulary.
Example:
- Original: "The movie was great."
- Augmented: "The film was excellent."
Random Insertion or Deletion
Words are randomly inserted or removed without changing the core meaning, forcing the model to learn context better.
Back Translation
Translate a sentence to another language and back to English (or the original language). It changes the structure while preserving meaning.
Example:
- Original: "This product exceeded my expectations."
- Back Translated: "I was surprised by how well this product performed."
Audio Data Augmentation Techniques
In speech or sound recognition, these techniques help simulate real-world audio environments:
Time Stretching
Changes the speed of the audio without altering the pitch.
Pitch Shifting
Changes the pitch of the voice or sound slightly.
Background Noise Addition
Simulates different acoustic environments by mixing in background noise like traffic, café sounds, or static.
These methods improve robustness in voice assistants, speech recognition, and music genre classification.
Each of these data augmentation techniques plays a crucial role in improving model performance, especially when data is scarce or imbalanced.
Real-World Examples of Data Augmentation in Deep Learning
Data augmentation isn’t just a theoretical concept — it’s actively used across industries to train powerful, real-world deep learning models. Let’s explore some practical applications where data augmentation makes a massive difference.
1. Medical Imaging (Healthcare)
Challenge: Medical datasets like X-rays or MRIs are limited and sensitive due to patient privacy.
Solution: Augmentations like rotation, flipping, zooming, and contrast adjustments allow models to learn from limited samples while detecting conditions like tumors, fractures, or organ abnormalities more accurately.
- Use Case: Classifying lung conditions in chest X-rays with augmented datasets improves diagnostic accuracy, especially in rare disease categories.
2. Self-Driving Cars (Autonomous Vehicles)
Challenge: Models must recognize pedestrians, road signs, and obstacles under varying weather, lighting, and angles.
Solution: Techniques such as brightness shifts, random occlusion (simulating obstructions), and motion blur are used to simulate real-world driving scenarios.
- Use Case: Tesla and Waymo use data augmentation in training models for better decision-making on roads.
3. Natural Language Processing (Chatbots & Translators)
Challenge: Language models need to generalize across grammar variations, synonyms, and noisy input.
Solution: Text augmentation techniques like synonym replacement, back translation, and typo simulation are used to build smarter conversational agents and translation systems.
- Use Case: Google Translate and Duolingo use these techniques to train multilingual NLP models efficiently.
4. Voice Assistants (Speech Recognition)
Challenge: Models must handle various accents, speeds, and background noises.
Solution: Augmenting voice data with pitch shifts, background noise, and time stretching improves performance across environments.
- Use Case: Alexa, Siri, and Google Assistant use augmented audio data for better voice recognition accuracy in noisy or echo-prone areas.
5. E-commerce & Retail (Product Recommendations)
Challenge: Limited labeled data for new products or user behavior.
Solution: Augmented image and text data help models recognize products, detect fake reviews, and personalize recommendations.
- Use Case: Amazon and Flipkart use augmented images for better visual search and smarter recommendation engines.
These examples show how data augmentation isn't just about boosting accuracy — it’s about building robust, scalable, real-world AI solutions.
Also Read: What is LLM? A Complete Guide to Large Language Models in AI and Generative AI
Best Practices and Tools for Applying Data Augmentation
While data augmentation can significantly boost model performance, it’s important to apply it thoughtfully. Here are some best practices and tools to help you get the most out of data augmentation:
Best Practices
1. Preserve Labels
Ensure that augmentations do not change the meaning of the data. For example, rotating an image of the number "6" could turn it into a "9" — which would confuse a digit classifier.
2. Apply Randomization Carefully
Random augmentations increase data diversity but should stay within logical limits. For example, a horizontal flip makes sense for a dog, but not for text or directional signs.
3. Don’t Overdo It
Too much augmentation can create unnatural data. Find a balance between diversity and realism.
4. Use Augmentation in Training, Not Testing
Always apply data augmentation only during training. Your validation and test sets should reflect real, unmodified data to measure performance accurately.
5. Combine Multiple Techniques
Sometimes, chaining augmentations — like rotating + adding noise — leads to better results than using them in isolation.
Popular Tools and Libraries
You don’t need to build augmentation from scratch — powerful libraries already exist for every type of data:
For Images
- TensorFlow/Keras ImageDataGenerator
- Albumentations – Fast and flexible image transformations.
- Torchvision (PyTorch) – Built-in transforms for image pipelines.
- imgaug – Great for complex pipelines including geometric and pixel-level changes.
For Text
- NLPAug – Covers synonym replacement, contextual word embeddings, and more.
- TextAttack – Designed for augmenting and attacking NLP model
- EDA (Easy Data Augmentation) – Simple Python implementation for common text techniques.
For Audio
- Torchaudio – Audio transforms for PyTorch users.
- Audiomentations – Inspired by Albumentations, designed for speech/audio augmentation.
- WavAugment – Lightweight and effective for speech data.
Using the right combination of techniques and tools ensures that your model learns from rich, meaningful variations in data without being confused by noise.
Conclusion
In the world of deep learning, more data often means better performance — but collecting massive datasets is expensive, time-consuming, and sometimes impossible. That’s where data augmentation in deep learning becomes a game-changer.
By applying thoughtful transformations to existing data, we can:
- Improve model accuracy and generalization
- Overcome data scarcity and class imbalance
- Simulate real-world variations without needing new data
Whether you're working on images, text, or audio, mastering the right data augmentation techniques in deep learning can dramatically enhance your model’s robustness — especially when resources are limited.
With tools like Albumentations, NLPAug, and Torchaudio, implementing augmentation is easier than ever. And with best practices in mind, you’ll be able to train smarter models that perform well not just in the lab, but in the real world too.
Ready to transform your AI career? Join our expert-led courses at SkillCamper today and start your journey to success. Sign up now to gain in-demand skills from industry professionals. If you're a beginner, take the first step toward mastering Python! Check out this Full Stack Computer Vision Career Path- Beginner to get started with the basics and advance to complex topics at your own pace.
To stay updated with latest trends and technologies, prepare specifically for interviews, make sure to read our detailed blogs:
How to Become a Data Analyst: A Step-by-Step Guide
How Business Intelligence Can Transform Your Business Operations