In recent years, deep learning has revolutionized the way machines perceive and interpret data—enabling breakthroughs in image recognition, natural language processing, speech synthesis, and more. However, building deep learning models from scratch often requires massive datasets, extensive computational resources, and significant training time. This makes it impractical for many real-world scenarios, especially when labeled data is scarce or expensive to obtain.
That’s where transfer learning in deep learning comes in.
By leveraging knowledge gained from one task and applying it to a different but related task, transfer learning allows models to achieve high performance with much less data and training. Instead of starting from zero, we build upon the foundation of pre-trained models—those trained on large datasets like ImageNet or text corpora like Wikipedia—to solve new challenges efficiently and effectively.
This article will guide you through what is transfer learning in deep learning, how it works, and why it's a powerful technique for accelerating AI development. You’ll also see a practical transfer learning deep learning example and learn how to apply this strategy in your own projects.
Also Read: What is Gradient Descent in Deep Learning? A Beginner-Friendly Guide
What is Transfer Learning in Deep Learning?
Transfer learning is a machine learning technique where a model developed for one task is reused as the starting point for a model on a second, related task. In the context of deep learning, it means taking a pre-trained neural network—trained on a large benchmark dataset—and adapting it to a different problem that may have far less data.
To break it down simply: rather than training a neural network from scratch, which requires a lot of data and computational power, transfer learning allows us to "transfer" the learned features (like edges, textures, or abstract patterns) from a large, well-trained model to a smaller, task-specific one.
For example, a model trained to recognize animals in photographs can be reused to identify skin diseases, since both tasks involve understanding visual patterns in images.
Why Transfer Learning Works
Deep learning models learn features hierarchically—starting from low-level patterns like edges in early layers to high-level abstract features in deeper layers. These early and mid-level features are often generic and reusable across tasks, which is why transfer learning can be so effective.
When to Use Transfer Learning
You should consider transfer learning when:
- You don’t have a large labeled dataset.
- You want faster training time.
- You’re working on a problem similar to one that has already been solved using a pre-trained model.
It is widely used in:
- Image classification
- Object detection
- Sentiment analysis
- Speech recognition
- Medical diagnosis
How Transfer Learning Works
Transfer learning in deep learning can be applied in a few different ways depending on the size of your dataset and the similarity between the new task and the original task the model was trained on. Let's look at the two most common approaches:
1. Feature Extraction
In this method, you use the pre-trained model as a fixed feature extractor. Here's how it works:
- You remove the final classification layer of the pre-trained model.
- You keep the rest of the model's layers "frozen" (non-trainable).
- You add your own custom classifier layer at the end.
- You train only the new classifier on your dataset.
Use this when you have a small dataset and your new task is similar to the original task.
2. Fine-Tuning
Fine-tuning takes it a step further:
- You start with a pre-trained model.
- You unfreeze some of the top layers (the deeper, task-specific layers).
- You train these layers along with your own classifier on the new dataset.
Use this when you have a moderately sized dataset and want to adapt the model more deeply to your task.
Also Read: Everything You Need To Know About Optimizers in Deep Learning

Layer Freezing and Unfreezing
“Freezing” means keeping the weights of a layer unchanged during training. You typically freeze the early layers (which learn general patterns) and fine-tune the later layers (which learn task-specific patterns). This balances learning efficiency with task customization.
Transfer learning gives you flexibility—you can start with a ready-made model and either just plug in your data or fine-tune the model to your exact needs.
Transfer Learning Deep Learning Example
Let’s walk through a practical transfer learning deep learning example to understand how it’s applied in real-world scenarios.
Example: Image Classification Using ResNet50
Imagine you're building a model to classify images of flowers into five categories—roses, daisies, sunflowers, tulips, and dandelions. You only have a few hundred labeled images for each class, which isn't enough to train a deep convolutional neural network (CNN) from scratch.
Instead, you use ResNet50, a popular pre-trained model trained on ImageNet (which contains over 1 million images across 1,000 categories).
Here's how transfer learning would work:
- Load the pre-trained ResNet50 model, excluding its top classification layer.
- Freeze the base layers, so they retain their learned weights.
- Add your own dense layer (with softmax activation) to classify the five types of flowers.
- Train the model on your flower dataset—only the new layers are updated.
This way, you're using ResNet50’s powerful ability to detect patterns like edges, textures, and shapes without needing to train it from scratch.
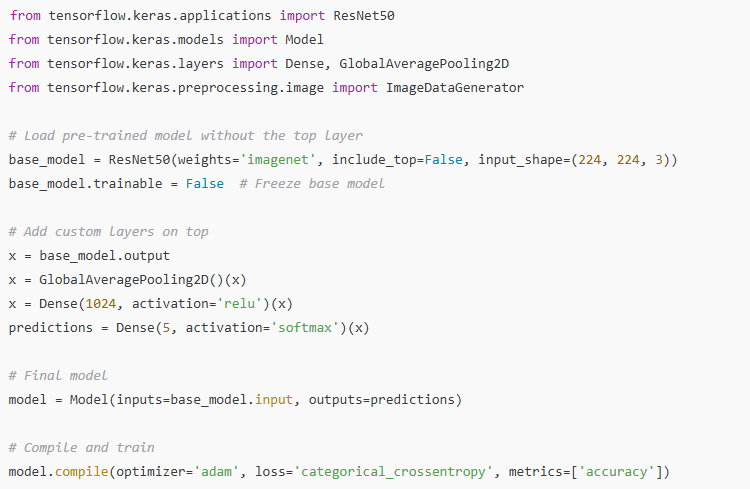
Real-World Applications of Transfer Learning
- Medical Imaging: Using ImageNet-trained models to detect tumors in X-rays or MRIs.
- Natural Language Processing (NLP): Fine-tuning BERT for sentiment analysis or question answering.
- Voice Assistants: Transferring knowledge from large speech datasets to new, domain-specific tasks.
- Retail: Using pre-trained vision models to detect damaged products or monitor shelf space.
- Medical Imaging: Using ImageNet-trained models to detect tumors in X-rays or MRIs.
Also Read: How Computer Vision Is Transforming Industries: Examples from Healthcare to Retail
Benefits of Transfer Learning in Deep Learning
Transfer learning isn’t just a trendy buzzword—it's a game-changer in making deep learning more accessible, efficient, and practical. Let’s look at the key benefits:
1. Faster Training
Since most of the model has already learned general features, you only need to train a small part (often just the classifier). This dramatically reduces training time, allowing you to build and iterate on models quickly.
2. Requires Less Data
Deep learning models typically need large labeled datasets. But with transfer learning, you can achieve high accuracy even with smaller datasets, which is ideal for domains like medical imaging or niche business problems where labeled data is scarce.
3. Leverages Pre-Learned Knowledge
Why reinvent the wheel? Pre-trained models already know how to detect edges, textures, and abstract features. Transfer learning allows you to reuse that learned knowledge, so your model starts smart rather than clueless.
4. Applicable Across Domains
Whether you're working in healthcare, finance, retail, or language processing, transfer learning deep learning techniques are flexible and can be adapted to various tasks—from image classification to text generation.
5. Saves Cost and Compute Resources
Training large models from scratch can be expensive, especially in cloud environments. Transfer learning lets you achieve good performance with lower computational costs, making deep learning accessible even to smaller organizations and individual developers.
6. Boosts Model Performance
In many real-world applications, transfer learning not only speeds up the process but also leads to higher accuracy, especially when the new task is related to the one used for pre-training.
Also Read: What is LLM? A Complete Guide to Large Language Models in AI and Generative AI
Conclusion
In a world where data is vast but not always labeled—and where computational resources can be expensive—transfer learning in deep learning offers a powerful shortcut. It lets us tap into the rich knowledge of pre-trained models and apply that to new, often smaller or specialized, tasks with great success.
Whether you're trying to classify medical scans with limited data or build a text classifier for a niche industry, transfer learning allows you to start strong, save time, and still achieve impressive results. It has become an essential technique in the modern deep learning toolkit, bridging the gap between research and real-world applications.
From image recognition to language models, the versatility of transfer learning ensures that you don’t always have to start from zero. Instead, you build on the shoulders of already-trained giants—fast-tracking your path to innovation.
Ready to transform your AI career? Join our expert-led courses at SkillCamper today and start your journey to success. Sign up now to gain in-demand skills from industry professionals. If you're a beginner, take the first step toward mastering Python! Check out this Full Stack Computer Vision Career Path- Beginner to get started with the basics and advance to complex topics at your own pace.
To stay updated with latest trends and technologies, to prepare specifically for interviews, make sure to read our detailed blogs:
How to Become a Data Analyst: A Step-by-Step Guide
How Business Intelligence Can Transform Your Business Operations