Full Stack MLOps Career Path
About This Course
- Gain a thorough understanding of MLOps concepts and practices, enabling efficient management and deployment of ML models.
- Master techniques for collecting, storing, versioning, and validating data to ensure high data quality and integrity.
- Learn to track experiments, tune hyperparameters, and version models to develop high-performing ML solutions.
- Understand how to use containerization and orchestration tools to deploy models in scalable and reliable ways.
- Implement CI/CD pipelines to automate testing, validation, and deployment of models, ensuring continuous improvement.
- Set up systems to monitor and log model performance, detecting issues proactively and maintaining model accuracy.
- Ensure ethical AI practices and compliance with regulatory standards, maintaining transparency and accountability.
- Apply MLOps principles in a real-world project, demonstrating practical skills and readiness for industry challenges.
- Kubeflow: Simplifies deploying ML workflows on Kubernetes, supporting all major ML frameworks.
- MLflow: Manages the ML lifecycle, including experimentation, reproducibility, and deployment with components like Tracking and Model Registry.
- TFX (TensorFlow Extended): Provides end-to-end ML pipeline management, from data ingestion to model deployment
- DVC (Data Version Control): Tracks large files, datasets, and models, ensuring reproducibility and collaboration.
- Seldon: Deploys, scales, and monitors ML models in production, offering features like canary deployments and drift detection.
- Docker: Containerizes ML applications for consistent environments and easier deployment across different platforms.
- Streamlit: An open-source app framework for creating custom ML tools and data science dashboards with minimal effort.
- Gradio: Allows quick creation of interactive demos and user interfaces for ML models, facilitating easy sharing and testing.
- Stakeholder Alignment: Ensure project goals align with business objectives through effective communication.
- Clear Documentation: Maintain comprehensive and accessible records of processes and workflows.
- Project Management: Plan, execute, and monitor MLOps initiatives to meet deadlines and standards.
- Data Presentation: Translate complex data insights into understandable information for non-technical audiences.
- Cross-Functional Collaboration: Coordinate seamlessly among data scientists, engineers, and business teams.
Introduction to MLOps offers a comprehensive overview of the core concepts and practices essential for managing and deploying machine learning models in production environments. It covers the unique aspects of MLOps, its benefits, and how it differs from traditional DevOps practices. Mastery of MLOps enables individuals to streamline ML workflows, enhance collaboration between data scientists and operations teams, and ensure the reliability and scalability of ML models, making it a crucial skill set for modern machine learning practitioners.
- 1.1 Overview of MLOps:
- MLOps (Machine Learning Operations) integrates machine learning with software engineering and operations to streamline the deployment, monitoring, and maintenance of ML models. MLOps ensures continuous integration, delivery, and automated deployment of ML models, fostering collaboration between data scientists and IT teams
- 1.2 MLOps vs. DevOps:
- While DevOps focuses on continuous integration and continuous delivery (CI/CD) for software applications, MLOps extends these principles to include data management, model training, validation, and deployment. MLOps deals with challenges specific to ML, such as versioning data and models, retraining models, and monitoring model performance.
- 1.3 Benefits of MLOps:
- Explore the advantages of adopting MLOps, including improved model reliability, scalability, and collaboration.MLOps enhances model reliability through continuous monitoring and automated testing, ensures scalability by leveraging cloud infrastructure and container orchestration, and fosters collaboration by integrating tools that streamline communication between data scientists and operations teams.
Data Management for ML focuses on the strategies and tools required for collecting, storing, versioning, and validating data, essential for ensuring data quality and integrity in machine learning projects. By mastering data management techniques, individuals can handle complex datasets, prepare data for model training, and maintain high standards of data quality, enabling them to derive actionable insights and build robust ML models.
- 1.1 Data Collection Methods:
- Learn methods for efficiently collecting data from diverse sources such as databases, APIs, and streaming platforms.Efficient data collection is essential for obtaining comprehensive datasets that adequately represent the problem domain. Techniques such as web scraping, API data extraction, and real-time data streaming are commonly used in industries like e-commerce for gathering customer behavior data, in finance for collecting market data, and in healthcare for capturing patient records from IoT devices.
- 1.2 Data Storage Systems:
- Understand the importance of choosing appropriate data storage systems for different types of datasets and use cases.Effective data storage is crucial for maintaining the integrity, availability, and scalability of large datasets. Relational databases are commonly used in enterprise applications for structured data storage, while NoSQL databases are preferred for unstructured or semi-structured data storage in applications like social media platforms and IoT systems. Cloud storage solutions offer flexibility and scalability, making them suitable for storing diverse datasets in industries ranging from retail to entertainment.
- 1.3 Data Versioning and Management:
- Understand the importance of versioning data to track changes and ensure reproducibility in machine learning workflows.Data versioning is critical for maintaining a historical record of dataset changes, facilitating collaboration, reproducibility, and experimentation in machine learning projects. Version control systems like Git are widely used in software development and data science for tracking changes to code and datasets. Data versioning practices are essential in research, healthcare, and autonomous systems development, where reproducibility and auditability are paramount for ensuring data integrity and model reliability.
Model Development and Training covers the essential processes of experiment tracking, hyperparameter tuning, and model versioning. These practices are crucial for developing high-performing and reproducible machine learning models. By mastering these techniques, individuals can streamline the model development process, ensure consistency, and improve model performance, ultimately leading to more effective and reliable ML solutions.
- 1.1 Experiment Tracking:
- is vital in industries like pharmaceuticals for documenting drug efficacy, dosage levels, and treatment protocols, ensuring reproducibility and collaboration among researchers.
- 1.2 Hyperparameter tuning:
- is extensively used in e-commerce for optimizing recommendation systems, enhancing customer satisfaction, and driving sales through improved product recommendations.
- 1.3 Model versioning:
- is crucial in autonomous vehicle development to maintain safety and performance under various driving conditions, allowing developers to track improvements, rollback changes, and ensure consistency across different iterations of the autonomous driving system.
Model Deployment focuses on the processes and tools required to move machine learning models from development to production environments. This module covers model serving architectures, containerization, and deployment strategies to ensure models can be quickly and reliably deployed. By mastering these techniques, individuals can ensure that ML models are scalable, maintain high performance, and are easily accessible for real-world applications.
- 1.1 Model Serving Architectures:
- Learn about different architectures for serving ML models in production.Model serving architectures find application in various real-world scenarios, such as online streaming platforms, where recommendation models are deployed to personalize content recommendations for users, ensuring a seamless and engaging user experience.
- 1.2 Containerization with Docker:
- Understand how to use Docker for containerizing ML models to ensure portability and scalability.Containerization with Docker is extensively utilized in healthcare systems for deploying medical imaging models, enabling healthcare providers to efficiently analyze and diagnose medical images while ensuring scalability and interoperability across different healthcare facilities.
- 1.3 Deployment Strategies::
- Explore various strategies for deploying models to production environments.Deployment strategies are essential in finance for deploying fraud detection models to detect and prevent fraudulent activities in real-time transactions, ensuring financial security and compliance with regulatory standards.
CI/CD for ML projects involves designing and implementing automated pipelines that streamline the testing, validation, and deployment of models. This module covers the necessary steps to maintain continuous integration and continuous deployment, ensuring models are always up-to-date and performing optimally. Mastery of CI/CD practices enables individuals to automate repetitive tasks, reduce deployment time, and improve model reliability.
- 1.1 CI/CD Pipelines for ML:
- Learn how to design and implement CI/CD pipelines specifically for machine learning projects.CI/CD pipelines for ML are crucial in industries like autonomous driving, where updates to perception models need to be seamlessly integrated into production vehicles to ensure safety and performance.
- 1.2 Automated Testing and Validation:
- Explore techniques for automating the testing and validation of ML models.Automated testing and validation techniques are extensively used in healthcare for validating medical diagnosis models, ensuring accuracy and reliability in clinical decision-making processes.
- 1.3 Continuous Deployment
- understand the process of continuously deploying updated models to production environments.Continuous deployment practices are vital in e-commerce for deploying product recommendation models, ensuring that users receive personalized recommendations based on the latest data, enhancing user experience and engagement.
Monitoring and Logging teaches techniques for setting up systems to continuously track model performance and log relevant metrics. Continuous monitoring is crucial for detecting issues such as model drift, performance degradation, and data inconsistencies. By mastering these techniques, individuals can ensure that models maintain high accuracy and reliability over time, enabling proactive maintenance and timely updates.
- 1.1 Monitoring Techniques:
- Learn how to set up monitoring systems to track model performance in production.Monitoring techniques are crucial in healthcare for monitoring patient risk prediction models, ensuring timely intervention and patient safety.
- 1.2 Logging Best Practices:
- Understand the best practices for logging model metrics and performance data.Logging best practices are widely applied in finance for logging trading algorithm performance metrics, enabling risk assessment and compliance monitoring.
- 1.3 Model Drift Detection:
- Explore methods for detecting and addressing model drift to maintain accuracy.Model drift detection is essential in manufacturing for detecting deviations in quality control models, ensuring product consistency and minimizing defects.
- Explore methods for detecting and addressing model drift to maintain accuracy.Model drift detection is essential in manufacturing for detecting deviations in quality control models, ensuring product consistency and minimizing defects.
Model Maintenance and Governance covers strategies for ongoing model maintenance, retraining, and ensuring ethical AI practices. This module emphasizes the importance of maintaining and updating models to keep them relevant and compliant with regulatory standards. By mastering these techniques, individuals can ensure that their ML models are ethical, transparent, and continuously improving.
- 1.1 Model Maintenance:
- Learn strategies for regularly maintaining and updating ML models to ensure ongoing performance.Model maintenance strategies are crucial in the retail industry for updating demand forecasting models to adapt to changing market trends and consumer behavior.
- 1.2 Retraining Models:
- Explore techniques for automating the retraining of models based on new data.Retraining models techniques are extensively used in telecommunications for automating the retraining of customer churn prediction models, ensuring accuracy and reliability in customer retention strategies.
- 1.3 Ethical AI and Compliance:
- Understand the principles of ethical AI and how to ensure compliance with regulatory standards.Ethical AI and compliance principles are vital in healthcare for ensuring patient privacy and confidentiality in medical diagnosis models, complying with HIPAA regulations and ethical guidelines.
- Understand the principles of ethical AI and how to ensure compliance with regulatory standards.Ethical AI and compliance principles are vital in healthcare for ensuring patient privacy and confidentiality in medical diagnosis models, complying with HIPAA regulations and ethical guidelines.
Make A Life-Changing Career Choice
Related Courses and Paths
Land Your Dream Job With
Full Placement Support
.avif)
Craft a Winning Resume
Nail Your Interview
Company Screening & Selection

What makes us different
POPULAR
Live Interaction
Self paced
Fee Structure
₹ 75,000
₹ 50,000
Curriculum & Course Materials
Live coding environment
AI-based learning platform
100+ hours of instruction
20+ assignments
10+ industry projects
Choose your industry specialization
Capstone projects
Live Classes
Flexible study options
Cancel anytime in first 7 days, full refund
Mentors
15+ hours of sessions with industry veterans & experts
Personalized mentorship by course instructors
Unlimited 1:1 doubt solving sessions
Career Support
Personalized placement assistance
1:1 mock interviews with industry experts
Soft-skills training module
Essential digital tools for digital workplace module
Interview preparation module
Masterclass on resume building & LinkedIn
Access to curated companies & jobs
POPULAR
Live Interaction
Self paced
Fee Structure
$599
$299
Curriculum & Course Materials
Live coding environment
AI-based learning platform
100+ hours of instruction
20+ assignments
10+ banking & finance case studies
Banking & finance domain focused curriculum
Capstone projects
Live Classes
Flexible study options
Cancel anytime in first 7 days, full refund
Mentors
15+ hours of sessions with industry veterans & experts
Personalized mentorship by course instructors
Unlimited 1:1 doubt solving sessions
Career Support
Personalized placement assistance
1:1 mock interviews with industry experts
Soft-skills training module
Essential digital tools for digital workplace module
Interview preparation module
Masterclass on resume building & LinkedIn
Access to curated companies & jobs
Frequently Asked Questions

Ready to become a Data Scientist that industry loves to hire? Apply Now.
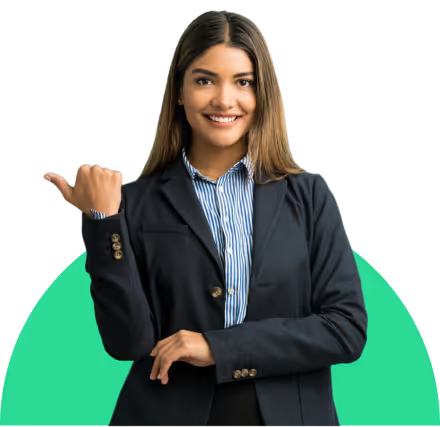