Data science — it’s the buzzword that’s been lighting up job boards and LinkedIn feeds for the past few years. It sounds exciting, doesn’t it? But with all the hype comes a whirlwind of misconceptions that can leave aspiring data scientists feeling overwhelmed or discouraged. You might have heard things like, “You need a PhD to get in,” or “All data scientists do is clean data.” Sound familiar?
The truth is, these myths can hold people back from exploring an incredibly dynamic and rewarding field. Whether you're just starting out or thinking about making a career switch, it’s time to clear the fog and get a real picture of what data science jobs are all about.
In this blog, we’re debunking the top 10 myths about data science jobs, so you can feel confident diving into this field with a fresh perspective. Ready to bust some myths? Let’s get started!
Myth #1: "You Need an Advanced Degree to Become a Data Scientist"
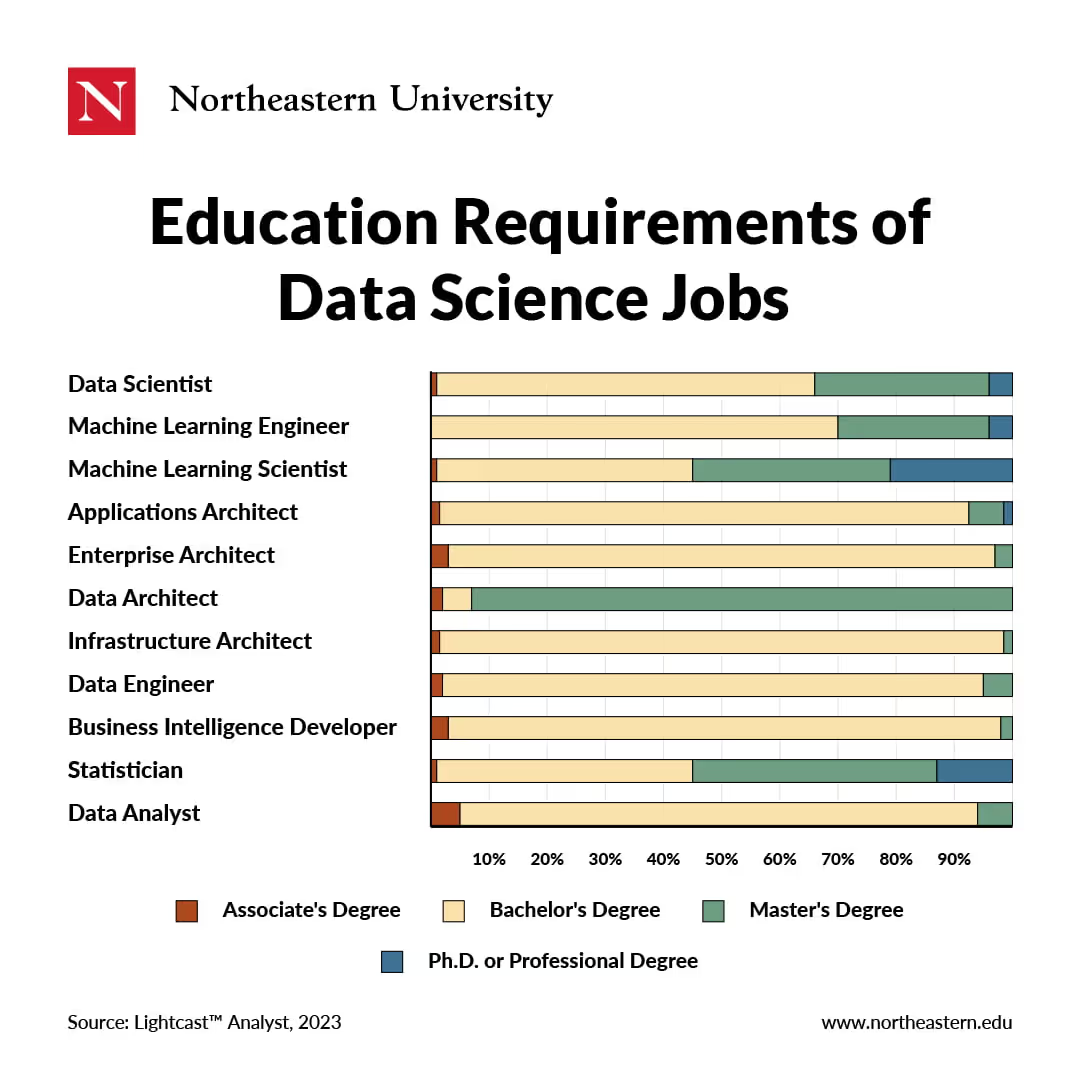
Here's a myth that keeps popping up: the belief that you need a master's degree or higher to make it in data science. It sounds intimidating, right? But let’s set the record straight — you don’t need a fancy diploma to dive into this field.
While an advanced degree can provide a deeper understanding of certain concepts or help with specialized roles, it's not the only way to get your foot in the door. In fact, many data scientists have entered the field with just a bachelor's degree or even through non-traditional routes like online courses, bootcamps, or self-study.
What truly counts is your ability to work with data, solve problems, and think analytically. Employers are more interested in what you can do with data than where you went to school. Building a strong portfolio with projects that showcase your skills, contributing to open-source work, or even participating in data science competitions can speak louder than a degree on your resume.
So, if you're passionate about data and willing to put in the effort to learn, don’t let the myth of needing an advanced degree hold you back. The door to a data science career is open — you just have to step through!
Myth #2: "Data Science Is All About Coding"
If you think data science is just about writing endless lines of code, you're not alone. Many people assume that data scientists spend all day in front of their screens, cranking out code like there's no tomorrow. But here’s the real deal: coding is just one piece of the puzzle.
Yes, knowing how to code is important. Python, R, SQL — these are essential tools in a data scientist’s toolkit. But being a data scientist is so much more than just coding. It’s about asking the right questions, understanding the business context, analyzing data, interpreting results, and — perhaps most importantly — communicating your findings to stakeholders who might not be so data-savvy.
Think of it this way: you could be the best coder in the world, but if you can’t explain your insights to your team or use those insights to drive real-world decisions, you’re missing the point of data science. The job is as much about storytelling and strategy as it is about syntax and scripts.
So, don’t get bogged down thinking you need to be a programming wizard to succeed in data science. Focus on developing a well-rounded skill set that combines coding with critical thinking, communication, and business acumen. That’s the true recipe for success in this field!
Myth #3: "Data Science Is Only for Math Geniuses"
Here’s a myth that stops a lot of people in their tracks: the idea that you need to be some kind of math prodigy to succeed in data science. If you’ve ever thought, “I’m not good enough at math to be a data scientist,” you’re definitely not alone. But let’s debunk this once and for all!
Sure, having a basic understanding of statistics and some mathematical concepts is essential — you can’t exactly run a regression analysis or build a machine learning model without it. However, you don’t need to be solving complex equations in your head or memorizing advanced calculus formulas daily. In fact, most of the heavy lifting is done by the software and tools you’ll use, like Python libraries (think NumPy or SciPy) or platforms like TensorFlow and Scikit-Learn.
The real challenge in data science isn’t about crunching numbers manually; it’s about knowing which methods to apply, interpreting the results correctly, and understanding the impact of those results on the business or problem you’re tackling. It's about asking the right questions and thinking critically about data.
So, if math isn't your strongest suit, don’t let that scare you away. Start with the basics, practice applying them, and remember: data science is less about complex math and more about using the right tools and approaches to make data-driven decisions.
Myth #4: "Data Scientists Work Alone"
Picture a data scientist hunched over a computer, alone in a dark room, buried in code — sounds like a scene from a tech thriller, right? But in reality, the image of the solitary data scientist couldn’t be further from the truth.
Data science is a team sport. Data scientists rarely work in isolation; they collaborate with a wide range of professionals, from data engineers and analysts to product managers, marketers, and executives. Why? Because data science is about solving problems that impact the entire organization, and those problems require input from multiple perspectives.
For example, you might work with data engineers to ensure you have clean, reliable data. Or you might team up with product managers to understand the business context behind the numbers. You could be presenting insights to non-technical stakeholders, helping them see the story behind the data and guiding strategic decisions.
In short, data scientists are often at the intersection of data, business, and communication. The ability to collaborate, share insights, and translate complex data into actionable recommendations is just as critical as any technical skill. So, if you’re a people person who loves working with diverse teams, data science might be even more up your alley than you thought!
Myth #4: "Data Scientists Work Alone"
Picture a data scientist hunched over a computer, alone in a dark room, buried in code — sounds like a scene from a tech thriller, right? But in reality, the image of the solitary data scientist couldn’t be further from the truth.
Data science is a team sport. Data scientists rarely work in isolation; they collaborate with a wide range of professionals, from data engineers and analysts to product managers, marketers, and executives. Why? Because data science is about solving problems that impact the entire organization, and those problems require input from multiple perspectives.
For example, you might work with data engineers to ensure you have clean, reliable data. Or you might team up with product managers to understand the business context behind the numbers. You could be presenting insights to non-technical stakeholders, helping them see the story behind the data and guiding strategic decisions.
In short, data scientists are often at the intersection of data, business, and communication. The ability to collaborate, share insights, and translate complex data into actionable recommendations is just as critical as any technical skill. So, if you’re a people person who loves working with diverse teams, data science might be even more up your alley than you thought!
Myth #5: "AI Will Replace Data Scientists"
Ah, the classic fear: “AI is coming for my job!” With AI and automation advancing rapidly, it’s easy to assume that data scientists will soon be out of work, replaced by machines that can crunch numbers faster and smarter. But let’s pump the brakes on this one.
While AI tools and automated machine learning platforms are making some tasks more efficient, they’re not replacing data scientists — they’re empowering them. Think of AI as your helpful assistant, taking over repetitive tasks like data cleaning, basic analysis, or model tuning, so you can focus on the more strategic and creative parts of your job: designing experiments, exploring data patterns, interpreting results, and generating insights that drive business decisions.
Moreover, AI and machine learning models still need human oversight. Data scientists are the ones who understand the nuances of the data, know how to ask the right questions, and can identify bias or errors in models. So, instead of fearing AI, think of it as a powerful tool that extends your capabilities and makes your work even more impactful.
Myth #6: "Data Science Is Only for Tech Companies"
When you think of data science, do Google, Facebook, or Amazon come to mind? It’s a common misconception that data science is just for big tech companies, but the truth is, data science is everywhere! Today, almost every industry — from healthcare to finance, retail to agriculture — is tapping into the power of data to make better decisions.
For example, hospitals use data science to predict patient outcomes and optimize staffing, while retailers analyze purchasing patterns to personalize customer experiences. Even sports teams leverage data to improve player performance and strategy. The applications are virtually limitless, and data scientists are in demand across all sectors.
So, if you’re interested in data science but don’t see yourself working in Silicon Valley, no worries! The skills you develop as a data scientist are transferable to many industries. You can follow your passion, whether it’s in healthcare, education, sports, entertainment, or any other field.
Myth #7: "Data Scientists Only Do Data Cleaning"
Okay, let’s get one thing straight: data cleaning is a significant part of a data scientist’s job, but it’s far from the only thing they do! Yes, ensuring your data is clean and reliable is crucial — it’s the foundation of any good analysis. But the role of a data scientist goes way beyond that.
Once the data is prepped, data scientists dive into the fun stuff: building predictive models, designing experiments, visualizing data to uncover hidden patterns, and translating those patterns into actionable insights. They help organizations understand trends, forecast outcomes, and make informed decisions based on data.
So, while data cleaning might not be the most glamorous task, it's just a stepping stone to the more exciting parts of the job. And honestly, every great data scientist knows that good data hygiene is essential to unlocking those valuable insights!
Myth #8: "Data Science Is a Fad That Will Pass"
Some skeptics out there might say, “Data science is just a passing trend — it’ll die out soon.” But let’s be real: data science is not a fleeting buzzword; it’s a critical function that’s reshaping industries worldwide. As businesses increasingly rely on data-driven decisions, the demand for data science skills is only growing.
The truth is, data science is continuously evolving. New tools, techniques, and technologies emerge all the time, keeping the field dynamic and exciting. What started as a niche profession has now become a fundamental part of nearly every industry’s strategy, from optimizing operations to creating personalized customer experiences.
So, if you’re worried that a career in data science might be short-lived, think again. The data revolution is here to stay, and those who master data science will be at the forefront of driving innovation in the years to come.
Myth #9: "You Need to Know Every Programming Language"
Ever heard someone say, “You must know Python, R, SQL, Java, C++, and more to be a data scientist”? It sounds overwhelming, doesn’t it? But here’s the good news: you don’t need to know every programming language under the sun to succeed in data science.
What’s important is picking a few core languages and getting really good at them. For most data science tasks, Python and R are the go-to languages. Python, in particular, is favored for its versatility and a massive library of tools and packages tailored for data analysis, machine learning, and deep learning. Knowing SQL is also valuable for querying databases, but beyond that, it’s more about choosing the right tool for the job rather than knowing them all.
Remember, employers are more interested in your problem-solving skills, ability to handle data, and knowledge of core concepts than in the number of languages you can list on your resume. Focus on mastering a few key languages, and you'll be well on your way!
Myth #10: "Data Science Is All About Big Data"
The term “Big Data” often gets thrown around as if it’s synonymous with data science. While big data can be an important part of the picture, data science isn’t just about wrangling massive datasets. In fact, data science can be incredibly valuable even with smaller datasets.
The magic of data science lies in its ability to find insights, patterns, and trends from data of any size. Whether it’s a startup analyzing customer feedback from a few hundred surveys or a non-profit using data to optimize donation campaigns, data science can be applied to problems big and small. It’s less about the volume of data and more about how you use that data to make better decisions.
So, if you’re intimidated by the idea of working with massive datasets, don’t be. Data science is about curiosity, creativity, and problem-solving — skills that apply no matter the size of the data you're working with.
Conclusion: Dispelling the Myths
There you have it — the top 10 myths about data science jobs, officially busted! Data science is a diverse and dynamic field with opportunities for all kinds of talent. Whether you're a coding enthusiast, a creative problem solver, or someone who loves making data-driven decisions, there’s a place for you here.
Don’t let these myths hold you back from exploring a career that could be both exciting and rewarding. Keep learning, stay curious, and remember: the world of data science is as wide as your imagination allows it to be!
Feeling inspired? Check out some free data science resources or join a community of learners to start your journey today. Follow this blog for more insights and updates.
Lorem ipsum dolor sit amet, consectetur adipiscing elit. Suspendisse varius enim in eros elementum tristique. Duis cursus, mi quis viverra.