
Introduction
In the fast-paced world of finance, the role of a data scientist in finance is becoming indispensable. These professionals are no longer just behind-the-scenes analysts—they're the driving force behind some of the most innovative and strategic moves in the industry. From predicting the next market shift to personalizing financial services down to the individual level, data scientists are redefining how we think about money.
As Hal Varian, Chief Economist at Google, famously said, “The ability to take data—to be able to understand it, to process it, to extract value from it, to visualize it, to communicate it—that’s going to be a hugely important skill in the next decades.” This couldn’t be truer for the financial sector, where data-driven insights are transforming every aspect of the business.
In this blog, we’ll unpack the cutting-edge trends shaping the future of finance and uncover the unique opportunities for data scientists to make a real impact. If you're curious about where finance is headed and how data science is at the heart of it all, you're in the right place.
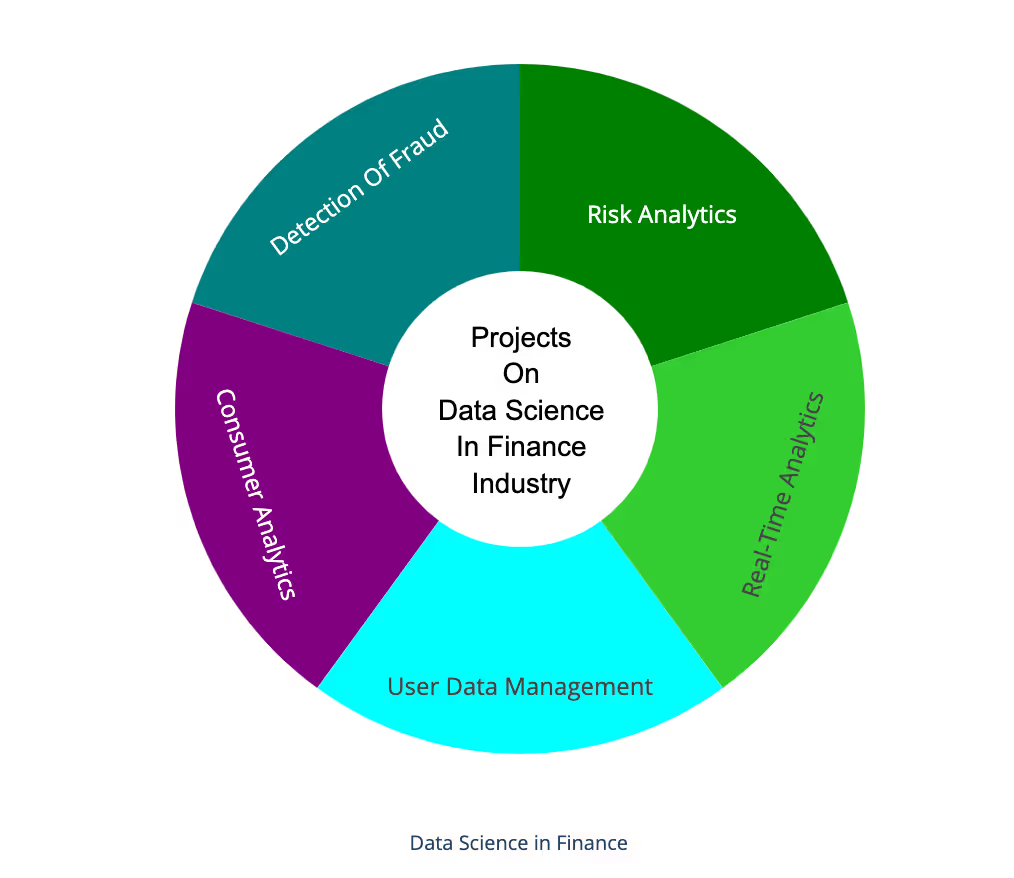
The Evolving Role of Data Scientists in Finance
Let’s be honest—being a data scientist in finance is not what it used to be. The role has transformed dramatically over the past few years, evolving from a back-office number cruncher to a key player in boardroom decisions. Today, data scientists are expected to wear multiple hats, blending their technical expertise with strategic insight to steer financial institutions through a complex and ever-changing landscape.
From Data Analysts to Strategic Advisors
In the early days, data scientists were mainly tasked with analyzing historical data and generating reports. They’d dig through spreadsheets, run some models, and hand over their findings to decision-makers. But today, the game has changed. Data scientists are now expected to be strategic advisors who don’t just analyze data but also interpret it in a way that directly informs business strategy. They’re the ones who can tell you not just what the numbers say, but what they mean for the future of your business.
Think about it: In a world where financial markets can turn on a dime, having someone who can predict trends, identify risks, and spot opportunities before they hit the mainstream is invaluable. That’s exactly what today’s data scientists do. They don’t just look at what happened—they anticipate what’s coming next, giving companies a competitive edge in an industry where timing is everything.
Key Responsibilities of Data Scientists in Finance
So, what exactly does a day in the life of a data scientist in finance look like? It’s a mix of deep analytical work and high-stakes decision-making. Here are some of the key responsibilities:
- Risk Modeling: Data scientists develop sophisticated models to assess and predict financial risks. Whether it’s credit risk, market risk, or operational risk, these models help financial institutions stay one step ahead of potential pitfalls.
- Algorithmic Trading: Ever wondered how those high-frequency trading algorithms work? Data scientists are behind the scenes, designing and refining the algorithms that make split-second trading decisions, often with millions of dollars on the line.
- Fraud Detection: In an era where cybercrime is on the rise, data scientists play a crucial role in detecting and preventing fraud. They use machine learning techniques to identify suspicious activities and flag potential threats before they can do any damage.
- Customer Analytics: Understanding customer behavior is key to delivering personalized financial services. Data scientists analyze vast amounts of customer data to uncover insights that help tailor products and services to individual needs, driving customer satisfaction and loyalty.
As you can see, the role of a data scientist in finance is as diverse as it is dynamic. It’s a career that demands not just technical skill but also a deep understanding of the financial world and the ability to think strategically. And as the industry continues to evolve, so too will the role of data scientists, offering even more exciting opportunities to shape the future of finance.
Current Trends in Financial Data Science

The financial world is in the midst of a data revolution, and being a data scientist in finance means you’re at the forefront of this transformation. With technology advancing at breakneck speed, the trends shaping the industry are as fascinating as they are game-changing. Let’s dive into some of the most impactful trends that are currently redefining the landscape of financial data science.
AI and Machine Learning: The New Powerhouse
AI and machine learning are driving massive shifts in finance, and these technologies are more than just buzzwords—they're fundamentally changing how financial institutions operate. From automating complex trading strategies to enhancing credit scoring models, AI is enabling data scientists to achieve outcomes that were once considered out of reach.
For instance, in algorithmic trading, AI-powered algorithms analyze market data in real time, executing trades at lightning speed based on patterns and predictions that no human could spot on their own. Or consider credit scoring: traditional models relied on a few key indicators, but with machine learning, data scientists can now incorporate a much broader range of data points—everything from social media activity to transaction history—creating more accurate and personalized credit profiles.
But it’s not just about processing data faster. AI and machine learning also help financial institutions better understand and predict customer behavior, leading to more tailored products and services. Whether it’s personalized investment advice via robo-advisors or real-time fraud detection, the applications are vast and expanding daily.
Big Data and Cloud Computing: Unlocking New Possibilities
We’ve all heard about big data, but what does it really mean for a data scientist in finance? In essence, big data refers to the massive volumes of structured and unstructured data generated every second—from transaction records to social media posts. The challenge and opportunity lie in making sense of it all.
Enter cloud computing. With the power of the cloud, financial institutions can store and process enormous amounts of data without the constraints of traditional infrastructure. This makes data more accessible and enables real-time analytics, giving data scientists the ability to provide insights faster and more efficiently.
Imagine being able to analyze global market trends as they happen or instantly assess the risk profile of a potential loan customer using data from thousands of sources. Big data and cloud computing make these scenarios a reality, opening new frontiers for innovation in finance.
Forecasting: The Crystal Ball of Finance
Forecasting has always been a cornerstone of financial decision-making, but with the advent of advanced data science techniques, it’s reaching new levels of sophistication. For a data scientist in finance, forecasting isn’t just about predicting the next quarter’s earnings—it’s about anticipating market trends, economic shifts, and customer behaviors with unprecedented accuracy.
Thanks to machine learning models and vast amounts of data, data scientists can now create highly accurate forecasts that consider a wide array of variables—from global economic indicators to granular customer data. These forecasts are invaluable for everything from investment strategies to risk management, allowing financial institutions to make informed decisions in an increasingly volatile market.
For example, predictive analytics can help banks anticipate loan defaults, enabling them to adjust interest rates or lending criteria proactively. Similarly, in asset management, forecasting models can predict market movements, helping investors optimize their portfolios and reduce risk.
In today’s fast-paced financial environment, the ability to forecast accurately can be a game-changer, giving institutions a competitive edge. And for data scientists, mastering the art of forecasting is not just a skill—it’s a necessity.
RegTech and Compliance: Navigating the Regulatory Maze
In a heavily regulated industry like finance, staying compliant is a top priority—and it's one where data scientists are making a significant impact. Enter RegTech, or regulatory technology, a field dedicated to using technology to ensure compliance with financial regulations.
For data scientists, RegTech presents both a challenge and an opportunity. The challenge is navigating the complex and ever-changing web of financial regulations. The opportunity lies in developing innovative solutions that not only ensure compliance but also streamline the process, reducing costs and risks for financial institutions.
For instance, data scientists are leveraging machine learning to automate compliance checks, flagging potential issues before they become problems. They’re also developing tools to monitor transactions for signs of money laundering or other illicit activities, helping financial institutions stay ahead of regulators.
As financial regulations become more stringent and complex, the demand for data-driven compliance solutions will only grow, making RegTech one of the most exciting areas for data scientists in finance today.
These trends—AI and machine learning, big data and cloud computing, forecasting, and RegTech—are just the beginning. The world of financial data science is evolving rapidly, and those who can stay ahead of the curve will find themselves in high demand, with opportunities to shape the future of finance in ways we’re only beginning to imagine.
Emerging Opportunities for Data Scientists in Finance
As the financial world rapidly evolves, data scientists in finance are finding themselves at the heart of some of the most exciting opportunities to drive change and innovation. Here’s a closer look at where these opportunities lie:
Sustainable Finance: Leading the ESG Revolution
With the surge in Environmental, Social, and Governance (ESG) investing, data scientists have a pivotal role in guiding sustainable financial decisions. For instance, they might analyze data on a company’s carbon emissions or social impact initiatives to help investors choose portfolios that align with their sustainability goals. This isn’t just about crunching numbers; it’s about making a real-world impact by steering capital towards companies that are doing good.
Example: A data scientist might develop a model that ranks companies based on their ESG performance, helping investment firms offer “green” portfolios to eco-conscious clients.
FinTech Innovation: Driving the Next Disruption
FinTech is reshaping how we think about money, and data scientists are the innovators behind the scenes. Whether it’s designing the algorithms that power peer-to-peer lending platforms or creating blockchain solutions for secure transactions, data scientists are at the cutting edge of financial technology.
Example: In the realm of blockchain, a data scientist might work on improving the efficiency of smart contracts, ensuring that transactions are automatically executed when conditions are met, without the need for intermediaries.
Personalized Financial Services: Crafting Tailored Experiences
Today’s customers expect financial services that are as unique as they are. Data scientists use customer data to create highly personalized financial products—like customized investment portfolios or loan offers that perfectly match an individual’s financial profile.
Example: Imagine a data scientist developing a model that predicts when a customer might need a mortgage based on their spending patterns and life events, then automatically presenting them with a personalized offer.
Risk Management and Cybersecurity: Protecting the Digital Realm
As finance goes digital, the risk of cyber threats has never been higher. Data scientists are crucial in identifying and mitigating these risks. They develop models to detect unusual activity, predict potential cyber attacks, and implement safeguards that protect financial institutions.
Example: A data scientist might design a machine learning model that analyzes transaction patterns in real-time, flagging any anomalies that could indicate fraud or a cyber attack, enabling a swift response to prevent financial loss.
These areas—sustainable finance, FinTech, personalized services, and risk management—are just a few of the exciting opportunities where data scientists can make a significant impact. The financial industry is ripe for innovation, and data scientists are uniquely positioned to lead the charge.
Skills Required for Success
Being a successful data scientist in finance requires a unique blend of technical expertise, domain knowledge, and soft skills. Here’s a breakdown of the key skills that will set you apart in this dynamic field:
Technical Skills: The Foundation of Your Toolkit
At the core of any data scientist’s skill set are the technical abilities that allow you to manipulate, analyze, and interpret vast amounts of data. In finance, these skills are particularly crucial because the stakes are high, and the data is complex.
- Programming Languages: Proficiency in programming languages like Python and R is essential. These languages are widely used for data analysis, modeling, and visualization. For example, Python’s libraries like pandas and NumPy are invaluable for financial data manipulation, while R is often preferred for statistical analysis.
- Machine Learning and AI: Understanding and applying machine learning algorithms is key, especially in areas like predictive analytics and risk modeling. Financial institutions rely on these models to forecast market trends, detect fraud, and optimize trading strategies.
- Data Visualization: The ability to present data in a clear, compelling way is crucial. Tools like Tableau, Power BI, and Matplotlib help data scientists translate complex data sets into visual insights that decision-makers can easily understand and act upon.
- Big Data Tools: Familiarity with big data technologies like Hadoop, Spark, and cloud platforms like AWS or Google Cloud is becoming increasingly important. These tools allow data scientists to process and analyze massive datasets quickly, which is a common requirement in finance.
Domain Knowledge: Understanding the Financial Ecosystem
Technical skills are vital, but they must be complemented by a deep understanding of the financial industry. Knowing how markets work, understanding financial instruments, and staying up-to-date with regulations are all part of the job.
- Financial Markets: A solid grasp of how financial markets operate is essential. This includes understanding market dynamics, asset classes, and the factors that influence prices. For instance, if you’re building a predictive model for stock prices, you need to understand how economic indicators and market sentiment can impact your predictions.
- Regulatory Compliance: The financial industry is heavily regulated, and being knowledgeable about these regulations is crucial. Whether it’s understanding GDPR for data privacy or the specifics of financial regulations like Dodd-Frank, being able to navigate this landscape is a must.
- Risk Management: Understanding the principles of risk management is also key. This includes being able to quantify and model different types of risks, such as credit risk, market risk, and operational risk, and integrating these considerations into your data-driven solutions.
Soft Skills: The Human Element
While technical and domain expertise are critical, soft skills are what enable you to translate your insights into actionable strategies and communicate effectively with both technical and non-technical stakeholders.
- Communication Skills: Being able to explain complex data concepts in simple, clear terms is vital. Whether you’re presenting your findings to executives or collaborating with other teams, clear communication ensures your insights lead to real-world decisions.
- Problem-Solving: Data science in finance often involves tackling complex, unstructured problems. A strong problem-solving mindset is essential to navigate these challenges, whether it’s figuring out how to clean messy data or developing a novel approach to predictive modeling.
- Critical Thinking: The ability to think critically about data, question assumptions, and validate your findings is crucial. In finance, where decisions can have significant consequences, being thorough and analytical in your approach is non-negotiable.
- Collaboration: Working well with others, whether it’s within a data science team or across departments, is key to success. Finance projects often require input from various stakeholders, and the ability to collaborate effectively ensures that your data-driven solutions are aligned with broader business goals.
In summary, success as a data scientist in finance requires a well-rounded skill set that combines technical expertise, deep financial knowledge, and strong soft skills. By mastering these areas, you’ll be well-equipped to navigate the complexities of the financial industry and drive meaningful impact through data science.
Challenges and Ethical Considerations
Working as a data scientist in finance is rewarding, but it comes with its own set of challenges and ethical dilemmas. Here’s a quick look at the key issues you’ll need to navigate:
Data Privacy and Security: Protecting What Matters
Handling sensitive financial data means privacy and security are top priorities. You’re responsible for ensuring that customer information is secure and used appropriately. It’s not just about following regulations like GDPR—it’s about respecting the trust that customers place in you.
Bias and Fairness: Keeping It Equitable
Algorithmic bias is a real risk in finance. Whether it’s in credit scoring or lending decisions, biased models can lead to unfair outcomes. Your job is to identify and reduce this bias, ensuring your models treat all users fairly. Ethical data science goes beyond the code; it’s about creating solutions that are just and equitable.
Regulatory Challenges: Staying Compliant
Finance is one of the most regulated industries, and staying compliant is a constant challenge. From data protection laws to market regulations, you need to be on top of it all. But it’s not just about ticking boxes—ethical responsibility means making sure your work aligns with the broader goals of financial regulation, like protecting consumers and maintaining market integrity.
Transparency and Accountability: Building Trust
As AI becomes more integral to finance, transparency is crucial. People need to trust that these systems are making fair decisions. Instead of relying on “black box” models, aim for transparency—choose explainable models where possible, and be ready to account for the decisions your models make. Trust in AI starts with the data scientists who build it.
In summary, the challenges in financial data science are as much about ethics as they are about technology. By focusing on privacy, fairness, compliance, and transparency, you can help build a more trustworthy financial system.
Conclusion
The role of a data scientist in finance is more dynamic and impactful than ever before. As the financial industry continues to evolve, data scientists are at the forefront, driving innovation, uncovering new opportunities, and navigating complex challenges. From harnessing AI and big data to pioneering sustainable finance and FinTech, the contributions of data scientists are reshaping the future of finance.
However, with these opportunities come significant responsibilities. Ensuring data privacy, combating bias, adhering to regulations, and maintaining transparency are all critical to building a fair and trustworthy financial system. Success in this field requires not only technical expertise but also a strong ethical compass and a commitment to continuous learning.
Whether you're already in the field or considering a career in financial data science, there's no doubt that this is an exciting time to be part of the journey. The future of finance is data-driven, and data scientists are the ones leading the way.