As data science continues to expand its influence across industries, staying informed about the latest trends and technologies is crucial for professionals and organizations alike. In this detailed exploration, we dive into the transformative technologies shaping the future of the data world, its vast future scope, and the growing demand for skilled professionals.
1. Automated Machine Learning (AutoML)
Traditional machine learning requires extensive manual intervention, from data preprocessing to feature selection and model tuning. However, with the rise of Automated Machine Learning (AutoML), much of this process is becoming automated, reducing the time and expertise required to develop high-performing models.
Key Features of AutoML:
- Model Selection and Hyperparameter Tuning: AutoML automates the selection of the best-performing machine learning models and fine-tunes hyperparameters to achieve optimal performance.
- Feature Engineering: AutoML tools can automatically create new features, remove redundant ones, and handle missing data, which improves model accuracy without requiring manual feature engineering.
- Interpretability: Many AutoML platforms are focused not only on accuracy but also on creating interpretable models, which helps businesses understand how decisions are made.
Scope: AutoML democratizes machine learning, making it accessible to professionals without deep technical expertise. As businesses increasingly rely on data-driven insights, AutoML will enable a broader range of industries to adopt AI and machine learning. By reducing the need for highly specialized data scientists, AutoML will expand the future scope of data science across sectors such as finance, marketing, healthcare, and education and thereby there will be a huge data science demand in future.
2. Real-Time Data Processing and Analytics
The ability to analyze and act on data in real-time is becoming a competitive advantage for businesses. Real-time data processing refers to the continuous ingestion, analysis, and visualization of data as it is created. With the proliferation of IoT devices, mobile applications, and social media, the volume of real-time data has exploded.

Technologies Driving Real-Time Analytics:
- Stream Processing Platforms: Tools like Apache Kafka, Apache Flink, and Spark Streaming allow organizations to process data in real-time, handling millions of events per second.
- Cloud-Based Solutions: Major cloud providers (AWS, Google Cloud, Azure) offer real-time data pipelines that can scale dynamically, making it easier for organizations to deploy real-time analytics solutions without building complex infrastructure.
Use Cases:
- Fraud Detection: Financial institutions use real-time data analytics to detect fraudulent transactions instantly and prevent losses.
- Customer Experience: E-commerce platforms utilize real-time data to optimize the user experience by analyzing browsing behavior, providing recommendations, and personalizing offers in real-time.
Scope: The future of data science will see a significant focus on real-time data analytics, particularly in industries like finance, healthcare, retail, and telecommunications. As businesses shift toward more agile and responsive business models, the demand for data scientists skilled in real-time processing will increase.
3. Edge Computing
Edge computing refers to the practice of processing data closer to the source of data generation (such as IoT devices) rather than relying on a centralized data center or cloud. This reduces latency, saves bandwidth, and enables faster decision-making in real-time applications and will become an important technology among the data science demand in future.
Why Edge Computing Matters:
- Reduced Latency: In use cases like autonomous driving, even milliseconds matter. Processing data at the edge allows decisions to be made quickly, reducing the risk of accidents or system failures.
- Improved Security: Processing sensitive data locally at the edge reduces the need for transmitting large volumes of data over the internet, lowering the risk of cyberattacks.
- Cost Efficiency: By processing data at the edge, companies can reduce the cost of data transmission and cloud storage, leading to significant savings.
Scope: Edge computing is particularly relevant in industries like manufacturing, automotive, smart cities, and healthcare. The future of data science will increasingly involve the integration of edge computing to handle the data generated by the growing network of IoT devices. Data scientists with expertise in edge analytics and IoT will be in high demand as the need for real-time, localized data processing grows.
4. Ethical AI and Data Privacy
The rapid growth of AI and machine learning has brought significant concerns around the ethics of data use, model fairness, and privacy. Data science professionals must now navigate a landscape where ethical considerations are as important as technical proficiency.
Challenges in Ethical AI:
- Bias in AI Models: Machine learning models are only as good as the data they are trained on. If historical data contains biases, these biases can be perpetuated and even amplified by AI systems.
- Transparency and Explainability: As AI systems are increasingly used in high-stakes decisions (e.g., hiring, credit scoring, law enforcement), the need for transparent, interpretable models becomes critical. Black-box models, where decision-making processes are opaque, are facing increased scrutiny.
- Data Privacy: With regulations such as GDPR (Europe) and CCPA (California), organizations must ensure that they collect, store, and process data in compliance with legal standards. Privacy-preserving techniques like differential privacy and federated learning are gaining traction.
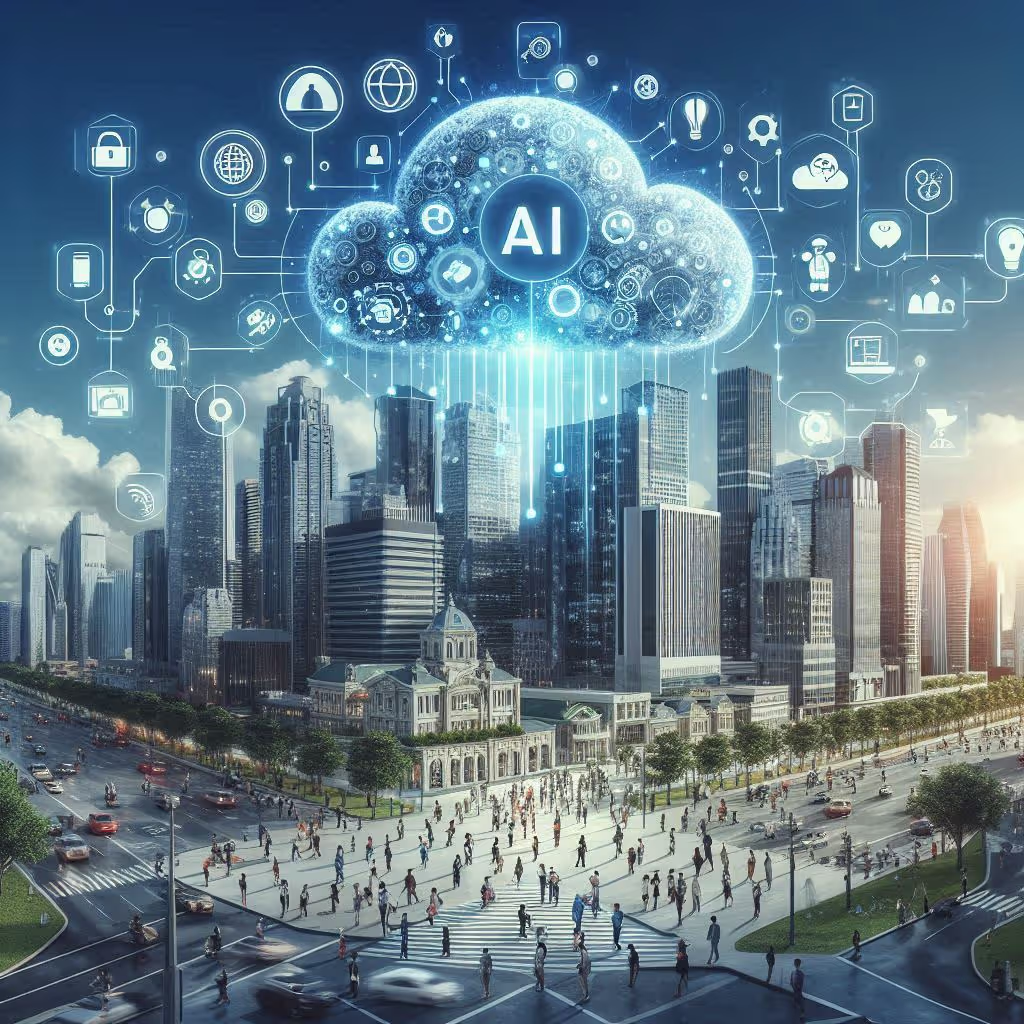
Scope: Ethical AI and privacy will be core components of the future years of data science. Organizations will require data scientists to not only build models but also ensure that they are fair, transparent, and compliant with legal standards. This will lead to a growing demand for professionals specialized in AI ethics and data privacy.
5. Quantum Computing
Quantum computing represents one of the most exciting frontiers in technology, promising to solve problems that are intractable with classical computers. In the context of data science, quantum computing could revolutionize areas such as optimization, encryption, and large-scale simulation.
How Quantum Computing Will Impact Data Science:
- Faster Algorithms: Quantum algorithms, like Shor's and Grover's algorithms, have the potential to solve problems in seconds that would take classical computers years or even centuries. For example, quantum optimization algorithms could dramatically improve the efficiency of supply chains, financial portfolios, or energy grids.
- Data Encryption: Quantum computing poses both a threat and an opportunity for cryptography. On one hand, it could break existing encryption schemes, but on the other hand, it will lead to the development of quantum-resistant cryptographic methods.
- Complex Simulations: Industries like pharmaceuticals and materials science can leverage quantum computing to simulate molecular structures, dramatically accelerating drug discovery and material design.
Scope: Though still in the research phase, quantum computing is set to become a critical component of industries that require complex problem-solving, such as finance, pharmaceuticals, and logistics. Data scientists with knowledge of quantum computing will be uniquely positioned to leverage this cutting-edge technology in the coming years.
6. Natural Language Processing (NLP) Advancements
Natural Language Processing (NLP), a subfield of AI that enables computers to understand and generate human language, has seen dramatic progress. Recent advancements in large language models like GPT (Generative Pre-trained Transformer) and BERT (Bidirectional Encoder Representations from Transformers) have revolutionized tasks such as text summarization, translation, and sentiment analysis.
Key NLP Applications:
- Chatbots and Virtual Assistants: NLP powers chatbots and virtual assistants, enabling companies to automate customer service while providing accurate, human-like responses.
- Sentiment Analysis: Businesses use NLP to gauge customer opinions on social media, reviews, and other text sources, providing actionable insights for marketing and product development.
- Document Analysis: In industries like law and finance, NLP models analyze contracts, reports, and other documents, extracting key information and automating workflows.
Scope: As unstructured data (text, voice, images) continues to grow, NLP will play a larger role in deriving insights from this data. Data scientists with NLP expertise will find increasing opportunities in industries like healthcare (analyzing medical records) and finance (extracting insights from reports).
7. Increased Demand for Specialized Data Roles
As the data science field matures, we are witnessing the rise of more specialized roles. Generalist data scientists are being complemented by experts in subfields such as data engineering, machine learning engineering, and data ethics.
Emerging Specialized Roles:
- Data Engineers: Responsible for building and maintaining the infrastructure that allows data to be collected, stored, and processed.
- Machine Learning Engineers: Focused on deploying and optimizing machine learning models in production environments.
- AI Ethicists: Ensure that AI and machine learning models are ethical, fair, and compliant with legal standards.
- Business Translators: Professionals who bridge the gap between technical teams and business units, ensuring that data-driven insights are actionable.
Scope: The rise of specialized roles will create more career opportunities within the data science ecosystem. As AI and machine learning continue to integrate into business processes, demand for professionals with deep expertise in these areas will increase, expanding the data science future scope.
8. Data Science in Sustainability
As global focus shifts toward sustainability, data science is playing an instrumental role in addressing environmental challenges. By analyzing large datasets related to climate, energy, and resources, data science can provide insights into optimizing processes and reducing environmental impact.
Sustainability Use Cases for Data Science:
- Energy Efficiency: Data scientists can analyze energy consumption patterns in buildings and factories to optimize energy use and reduce waste.
- Climate Change Modeling: By analyzing weather patterns, carbon emissions, and deforestation data, data science can contribute to predictive models that help in understanding and mitigating climate change.
- Supply Chain Optimization: Data science can help companies build more sustainable supply chains by optimizing logistics, reducing carbon footprints, and improving resource utilization.
Scope: With governments and businesses committing to sustainability goals, the demand for data scientists to drive environmental initiatives will increase. Data science in sustainability will be a vital area of growth in the coming years, particularly in industries such as energy, agriculture, and manufacturing.
Future Demand for Data Science Professionals
The demand for data science professionals is expected to continue growing rapidly. The data science future scope is being driven by several key factors:
- Explosion of Data: The sheer volume of data being generated by IoT devices, social media platforms, and digital transactions is fueling the need for data science professionals to derive actionable insights.
- AI and Machine Learning: As businesses increasingly integrate AI and machine learning into their operations, the demand for skilled data scientists will surge.
- Automation and Decision-Making: More companies are looking to automate decision-making processes, which requires advanced predictive models and data-driven strategies, creating further demand for data professionals.
Conclusion
The future years of data science is set to be shaped by a combination of cutting-edge technologies and societal demands. From the rise of AutoML and real-time analytics to the emergence of quantum computing and ethical AI, the field is poised for exciting advancements. As these trends take hold, the scope and demand for data science professionals will continue to grow, opening new opportunities across industries. For data enthusiasts, now is the time to hone your skills and prepare for a future where data will be at the heart of innovation and decision-making.
Ready to transform your AI career? Join our expert-led courses at SkillCamper today and start your journey to success. Sign up now to gain in-demand skills from industry professionals.
If you're a beginner, take the first step toward mastering Python! Check out this Fullstack Generative AI course to get started with the basics and advance to complex topics at your own pace.
To stay updated with latest trends and technologies, to prepare specifically for interviews, make sure to read our detailed blogs:
- Top 25 Python Coding Interview Questions and Answers: A must-read for acing your next data science or AI interview.
- 30 Most Commonly Asked Power BI Interview Questions: Ace your next data analyst interview.
- Difference Between Database and Data Warehouse: Key Features and Uses: A must read for choosing the best storage solution.
Top 10 NLP Techniques Every Data Scientist Should Know: Understand NLP techniques easily and make your foundation strong.