In today's data-driven world, data science has emerged as a powerful tool across various industries, and healthcare is no exception. The ability to extract insights from healthcare data is revolutionizing patient care, operational efficiency, and medical research. However, data science implementation in healthcare comes with its unique set of challenges. In this article, we will explore the opportunities and obstacles, while offering solutions to help healthcare organizations fully harness the power of data science.
__________________________________________________________________________
Healthcare generates vast amounts of data, from patient records and imaging to lab results and operational metrics. By leveraging this data, healthcare providers and researchers can improve diagnostics, personalize treatments, predict patient outcomes, and streamline hospital operations.
Current Applications of Data Science in Healthcare:
- Predictive Analytics: Data science enables hospitals and clinics to predict patient admissions, disease outbreaks, and medical complications. This helps in optimizing resources and enhancing preparedness.
- Personalized Medicine: Machine learning models analyze patient data, such as genetics, lifestyle, and medical history, to recommend tailored treatments. Personalized medicine can lead to more effective care plans and better patient outcomes.
- Medical Imaging: AI-powered models are increasingly being used to analyze medical images, such as X-rays, CT scans, and MRIs, for faster and more accurate diagnosis.
- Operational Efficiency: Data science helps streamline administrative processes, optimize staff scheduling, and reduce wait times, enhancing the overall patient experience.
- Drug Discovery: Pharmaceutical companies are using data science to accelerate drug discovery and clinical trials by analyzing vast datasets to identify potential drug candidates.

Challenges in Implementing Data Science in Healthcare
The potential of data science to revolutionize healthcare is undeniable. From predictive analytics to personalized medicine, data-driven solutions offer the promise of better care and more efficient operations. However, healthcare data comes with its unique set of challenges. Below, we delve deeper into each challenge, exploring its impact and offering solutions to overcome these barriers.
1. Data Privacy and Security
Healthcare sector data is among the most sensitive types of information, containing personally identifiable information (PII), medical histories, diagnostic results, and treatment plans. With increasing digitalization and cloud storage of healthcare records, the risks of data breaches have grown significantly.
- Impact: A breach of patient data can lead to serious consequences, such as identity theft, fraud, and violations of patient confidentiality. Moreover, regulatory frameworks like HIPAA (in the U.S.) impose stringent penalties for mishandling data in healthcare, which can result in legal action and loss of trust.
- Solution: To combat this, healthcare organizations must invest in advanced encryption technologies, multi-factor authentication, and regular security audits. Data anonymization can also play a crucial role by stripping out personally identifiable information while still allowing for analysis. Additionally, privacy-preserving technologies like federated learning, which allows machine learning models to train across decentralized data without actually moving the data, can be employed to mitigate the risk of exposure.
2. Data Silos and Fragmentation
One of the biggest barriers to effective data science applications in healthcare is the fragmented nature of healthcare related data. Hospitals, clinics, and research institutions often use different systems to store patient data, which creates data silos. This lack of interoperability between systems makes it difficult to compile comprehensive datasets for analysis.
- Impact: Fragmented data leads to incomplete patient profiles, impeding comprehensive analyses, and making it difficult to develop accurate predictive models or to optimize patient care pathways. It also complicates large-scale studies and research efforts that depend on unified datasets.
- Solution: The development of interoperability standards like FHIR (Fast Healthcare Interoperability Resources) can facilitate smoother data exchanges between disparate systems. Implementing Electronic Health Record (EHR) systems that comply with these standards can enable seamless sharing of patient data across institutions. Healthcare organizations must also prioritize investments in data integration platforms that pull information from various sources into unified, accessible formats.
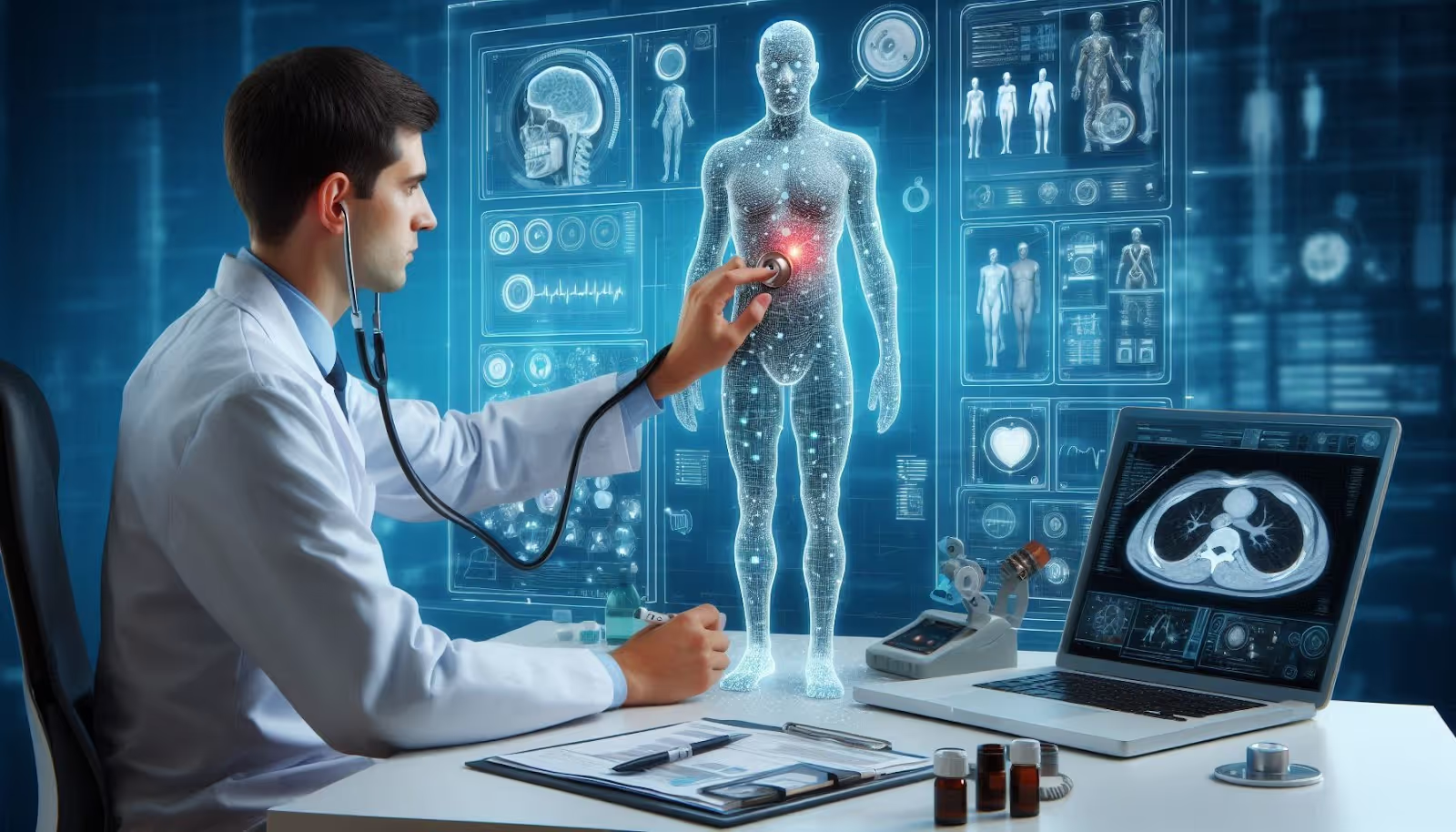
3. Data Quality and Inconsistency
The quality of healthcare related data is often compromised due to missing entries, inconsistent coding practices, and human errors in data entry. For instance, handwritten notes in medical records may not always be digitized accurately, and different institutions might use varying terminologies for the same medical conditions.
- Impact: Poor data quality can lead to inaccurate analysis and flawed predictive models. Inconsistent coding or missing data points might skew results, affecting clinical decision-making and research outcomes. This can further hinder personalized medicine efforts, as accurate, high-quality data is necessary for tailoring treatments.
- Solution: Data quality can be enhanced through rigorous data governance frameworks that include data cleaning protocols, standardized coding systems (such as ICD for medical diagnoses), and real-time validation tools to check for errors as data is entered. Artificial intelligence can also be applied to fill in missing values or correct discrepancies in large datasets, improving the integrity of the data.
4. Lack of Skilled Professionals
Implementing data science in healthcare requires professionals with expertise in both data science and healthcare domains. This dual skill set—technical expertise in data analytics and a deep understanding of clinical workflows—is difficult to find, leading to a talent shortage in the field of healthcare applications of data science.
- Impact: Without healthcare based data scientists, healthcare organizations struggle to translate data into actionable insights. Even when advanced AI models are developed, a lack of domain expertise can result in misinterpretation of the data, which can have direct consequences on patient care or lead to ineffective operational strategies.
- Solution: To address this shortage, institutions should invest in interdisciplinary education programs that combine healthcare, data science, and machine learning. Moreover, partnerships between academic institutions, healthcare organizations, and tech companies can foster training programs that create professionals who are adept in both fields. Encouraging collaboration between healthcare professionals and data scientists can also lead to cross-disciplinary learning, closing the gap over time.
5. Ethical Concerns and Bias in AI Models
Healthcare AI systems are not immune to bias, which can stem from skewed or incomplete training datasets. Bias in AI can arise from historical data that reflects societal disparities, leading to discriminatory outcomes, particularly against minority groups or underserved populations.
- Impact: Biased AI models can lead to inappropriate treatment recommendations, misdiagnoses, or even denial of care to certain patient demographics. For example, if a model is trained predominantly on data from one ethnic group, its predictive accuracy might suffer for other groups, leading to unequal healthcare outcomes.
- Solution: Addressing bias requires careful attention to how data is collected, curated, and used in AI models. Ensuring diversity in training datasets is crucial to developing fair and representative models. Regular audits for bias, along with the implementation of explainable AI (XAI) techniques, can help healthcare professionals understand how decisions are made and prevent bias from influencing clinical outcomes. Ethical oversight committees can also be established to monitor AI deployments and ensure fairness in decision-making.
6. Regulatory and Compliance Issues
Healthcare is one of the most heavily regulated industries, with strict rules governing the use, storage, and transmission of patient data. Navigating these regulations while implementing data science can be complex and time-consuming. Regulations such as HIPAA in the U.S., GDPR in Europe, and local health data laws globally require organizations to follow stringent rules regarding patient consent, data access, and data protection.
- Impact: Failure to comply with these regulations can result in severe financial penalties, legal liabilities, and a loss of trust from both patients and stakeholders. Regulatory hurdles can also slow down the deployment of data science tools, delaying potential benefits.
- Solution: Healthcare organizations should work closely with legal teams and data privacy experts to ensure compliance from the outset of any data science initiative. This includes creating comprehensive data governance frameworks, performing regular compliance audits, and educating staff about data protection laws. Additionally, ensuring patient consent is obtained when using their data for analytics is essential for staying within legal boundaries.
7. Integration with Existing Systems
Most healthcare providers rely on legacy IT systems that were not built with modern data science tools in mind. These systems often lack the flexibility and processing power required to support advanced analytics and AI solutions, making it difficult for hospitals and clinics to integrate new data science technologies.
- Impact: The inability to integrate modern tools with existing systems delays the adoption of innovative healthcare solutions, stifling opportunities for operational improvement and patient care enhancements.
- Solution: A gradual, phased approach to integration can minimize disruptions. Healthcare providers can start by deploying cloud-based platforms that offer greater flexibility and easier integration with legacy systems. These platforms also allow for scaling data science applications, making it easier to manage large datasets and AI models. Working with technology vendors to develop customizable solutions that fit the specific needs of healthcare organizations can also facilitate smoother transitions.
Overcoming Challenges: The Role of Healthcare Data Scientists
A healthcare based data scientist plays a critical role in addressing these challenges. They combine expertise in data analysis with a deep understanding of healthcare systems to design solutions that improve patient outcomes and streamline operations.
- Collaborating with Clinicians: Healthcare based data scientists work closely with doctors and medical staff to ensure that data science initiatives align with clinical needs and ethical standards.
- Ensuring Data Quality: They focus on data cleaning and validation to ensure that the insights generated are accurate and actionable.
- Navigating Regulations: By staying up-to-date with healthcare regulations, healthcare focused data scientists ensure that their data projects remain compliant while maximizing the potential of analytics.
Conclusion
The implementation of data science applications in healthcare presents a wealth of opportunities but also brings challenges that must be carefully navigated. By addressing issues related to data privacy, quality, interoperability, and regulatory compliance, healthcare organizations can overcome the hurdles and unlock the full potential of healthcare related data. With the right strategies in place, data science can revolutionize patient care, making it more personalized, efficient, and effective.
As healthcare focused data scientists continue to bridge the gap between data and medicine, the future of healthcare will undoubtedly be shaped by data-driven insights, leading to a healthier world.
Ready to transform your AI career? Join our expert-led courses at SkillCamper today and start your journey to success. Sign up now to gain in-demand skills from industry professionals.
If you're a beginner, take the first step toward mastering Python! Check out this Fullstack Generative AI course to get started with the basics and advance to complex topics at your own pace.
To stay updated with latest trends and technologies, to prepare specifically for interviews, make sure to read our detailed blogs:
- Top 25 Python Coding Interview Questions and Answers: A must-read for acing your next data science or AI interview.
- 30 Most Commonly Asked Power BI Interview Questions: Ace your next data analyst interview.
- Difference Between Database and Data Warehouse: Key Features and Uses: A must read for choosing the best storage solution.
Top 10 NLP Techniques Every Data Scientist Should Know: Understand NLP techniques easily and make your foundation strong.