Data science is an exciting field. It mixes coding, statistics and solving real-world problems. That’s why so many people are drawn to it, whether they're switching careers or adding it to their current skillset.
But while most online courses teach you Python, machine learning and how to boost model accuracy, they often leave out what it’s actually like to work as a data scientist in the real world.
This article covers 10 common mistakes beginners make that don’t usually show up in tutorials but can hold you back if you’re not careful.
Also read: How to Prepare for a Data Science Interview
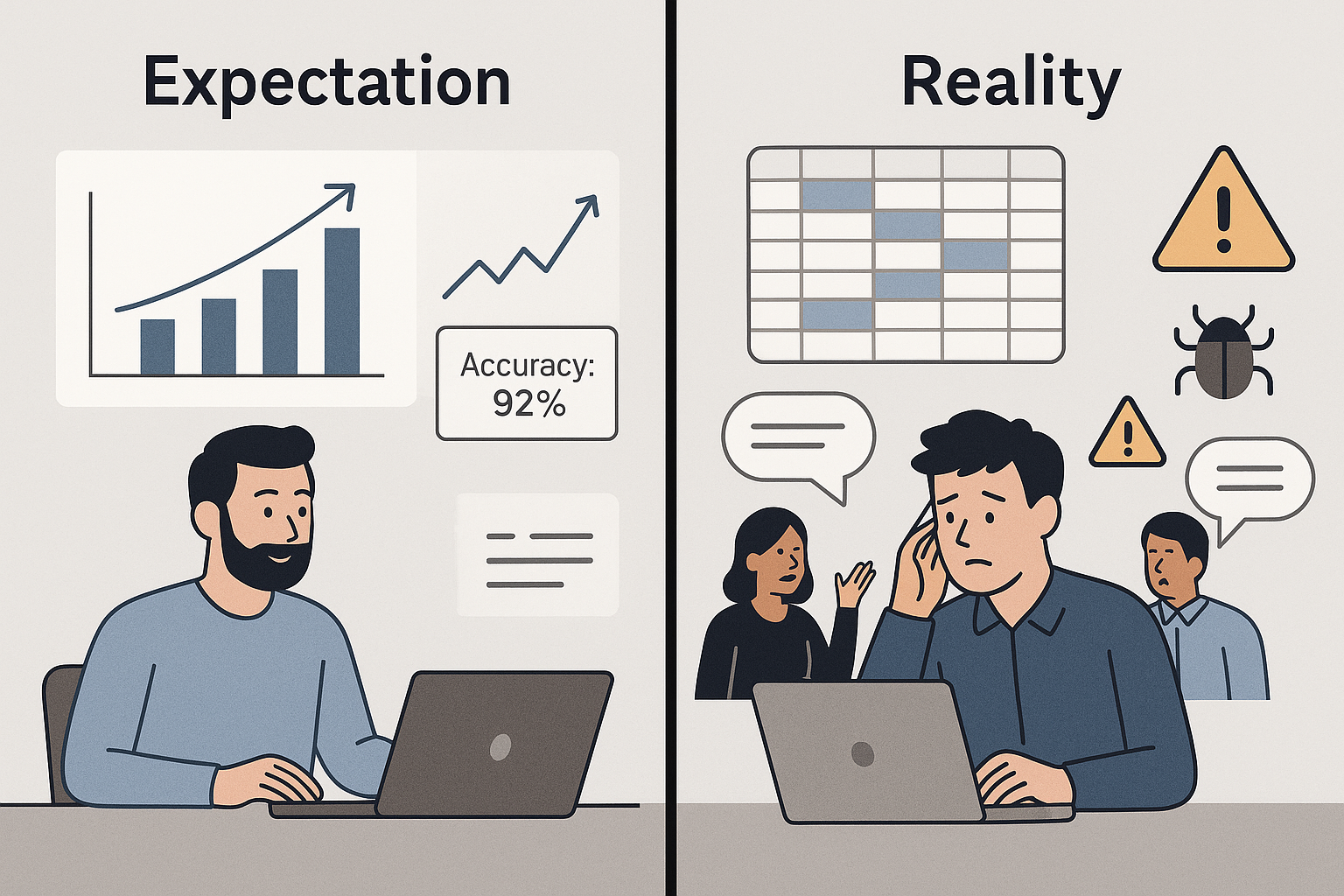
1. Ignoring Ethics and Privacy
When you’re excited about building models, it’s easy to forget about the ethical side of things. But data scientists often work with sensitive information like personal data, financial details, health records, etc.
If you don’t think about privacy, fairness or bias, your work could cause harm or even legal trouble.
Ask yourself:
- Did the user agree to share their data?
- Is my model treating certain groups unfairly?
- Are there hidden assumptions in the data?
In areas like healthcare or finance, getting this wrong isn’t just a mistake but can be serious.
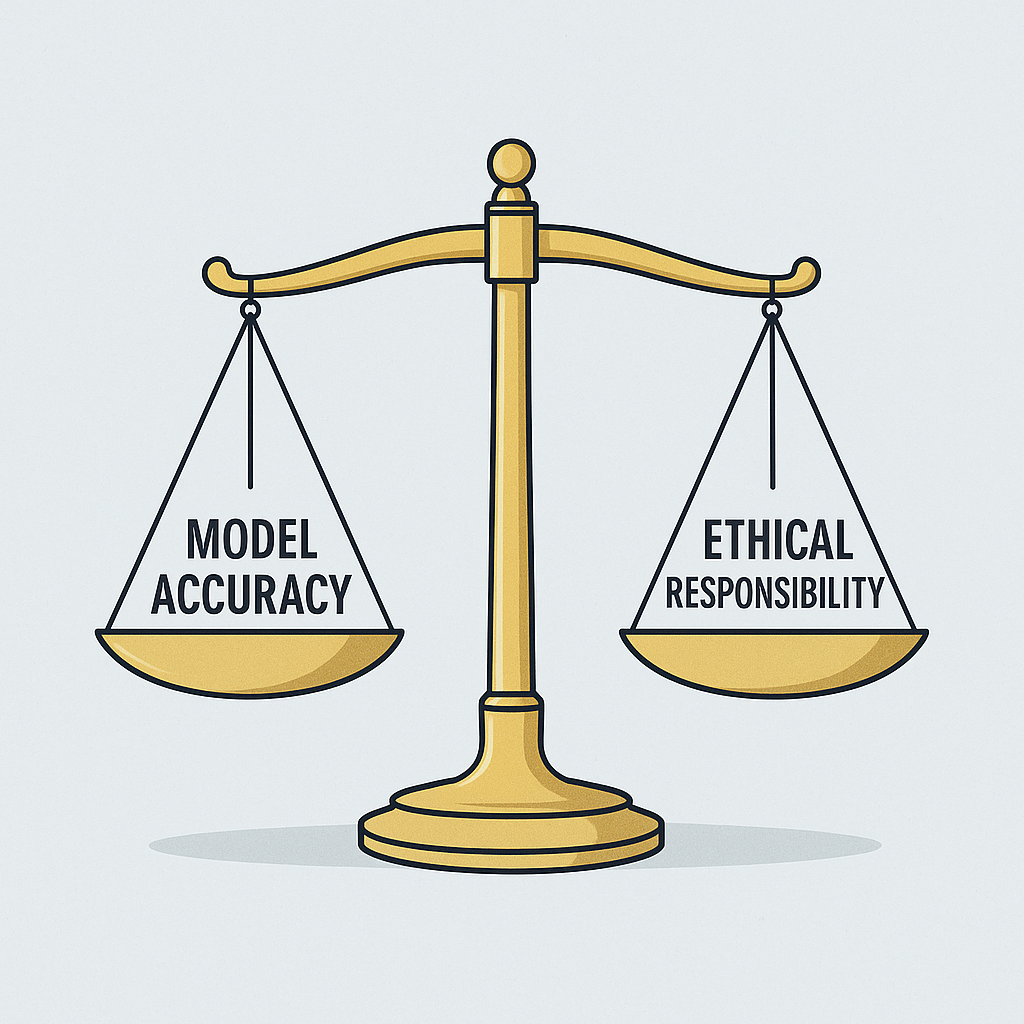
2. Not Understanding the Business Side
You could build the most accurate model ever, but if it doesn’t help the business, it doesn’t matter.
Always ask:
- What business problem am I solving?
- Who will use these insights, and what will they do with them?
- What outcome are stakeholders expecting?
Data science is about impact, not just accuracy. Knowing the bigger picture helps you build things that actually get used.
3. Weak Communication Skills
Being good at math and coding is great. But if you can’t explain your work clearly to non-technical teammates or clients, it won’t go very far.
Good data scientists:
- Keep their explanations simple and clear
- Connect data insights to business outcomes
- Work well with cross-functional teams (like marketing, product, or ops)
You don’t need to be a public speaker, just be clear and confident.
4. Forgetting About Deployment
A model isn’t done once it’s trained. In a real job, that model needs to be deployed, monitored and updated over time.
If you’ve never thought about:
- APIs
- Tools like Docker or FastAPI
- Version control or rollback options
Then you might get stuck when it’s time to move from “Jupyter Notebook” to “Live System.” Even learning the basics of deployment makes you way more valuable in a team setting.
5. Writing Messy, Hard-to-Read Code
When you're just starting out, it’s easy to write code that only you can understand. But in a real job, your code will be shared, reviewed and reused.
To make life easier for everyone:
- Use version control (Git)
- Write clean, modular code
- Add comments explaining why you did something
- Keep a list of required packages (with requirements.txt or environment.yml)
Your future self and your coworkers will thank you.
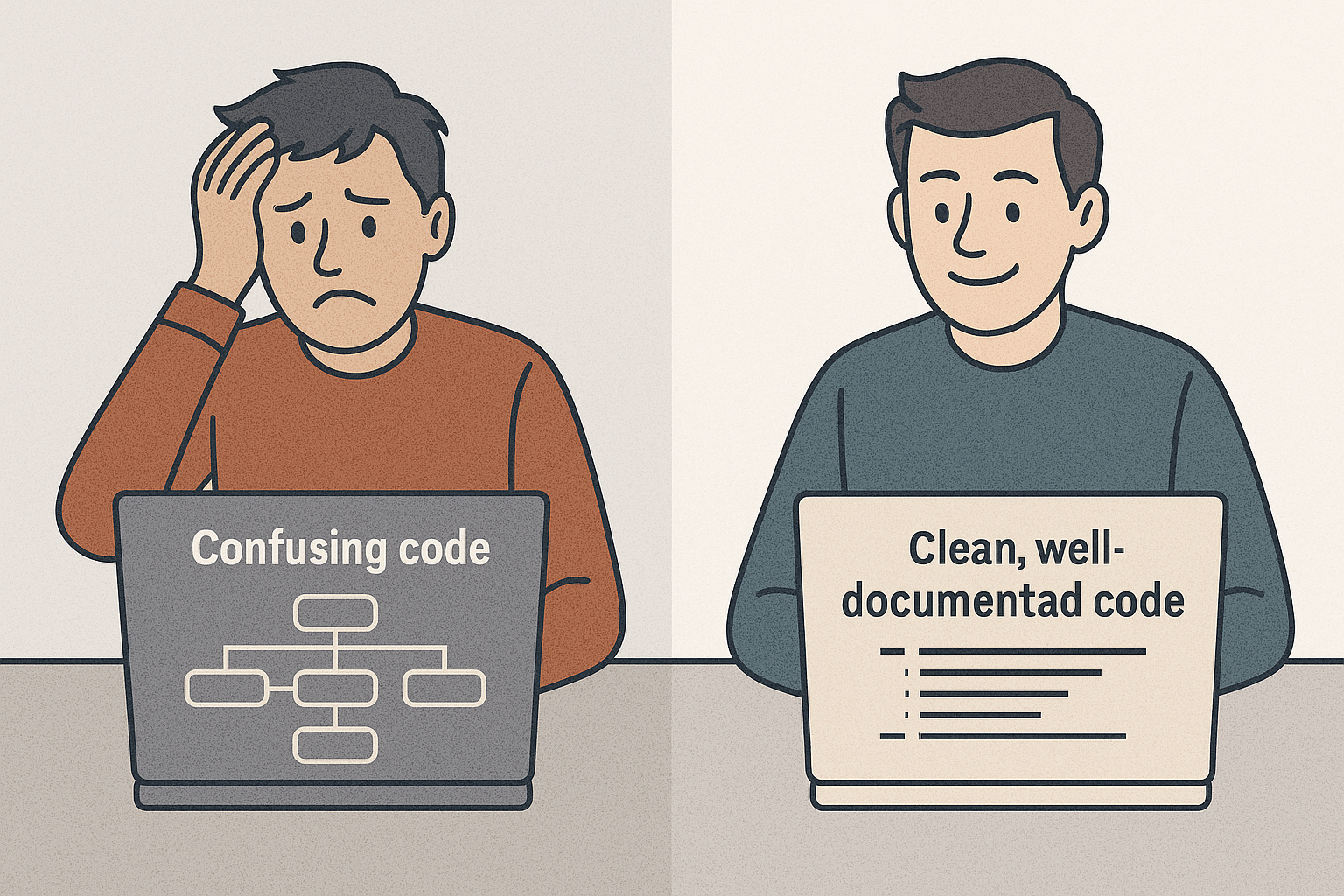
6. Thinking You’re Done Learning
Tech moves fast and data science is no exception. There’s always a new tool, library or approach around the corner.
But you don’t have to learn everything all at once. Instead:
- Follow trusted newsletters (like Towards Data Science or The Batch)
- Join communities like Kaggle or Reddit’s r/datascience
- Set aside a little time each week for learning
Make it a habit. Small, consistent learning beats cramming every time.
Also read: Top 10 YouTube Channels to Follow for Data Science in 2025
7. Relying Too Much on Tools Like AutoML or ChatGPT
Tools like AutoML, ChatGPT and others can speed up your workflow. But they’re helpers and not replacements for understanding.
If you only use tools without knowing how they work, you risk:
- Misunderstanding results
- Struggling to fix problems
- Being unprepared in job interviews
Use these tools to enhance your thinking, not replace it.
8. Avoiding Messy, Real-World Data
Many beginner projects use clean, easy datasets like Iris or Titanic. But in real life, data is messy.
You’ll face:
- Missing values
- Weird formats
- Duplicate entries
- Irrelevant or outdated features
Getting good at cleaning and preparing data is one of the most important skills you can develop. Look for projects with real-world data or better yet, work on open-source or volunteer data projects.

9. Skipping Feedback and Iteration
Some beginners think, “My model is done, I got 90% accuracy, let’s move on.”
But in reality, data science is iterative. You’re always refining, improving and learning.
To grow:
- Ask for feedback from mentors, peers or online communities
- Share early versions of your work for review
- Be open to revisiting your ideas as new data comes in
Feedback is a shortcut to better results and faster learning.
10. Trying to Learn Everything at Once
Data science is broad. Machine learning, data engineering, analytics, visualization, statistics, MLOps… it’s a lot.
If you try to learn it all, you’ll burn out.
Instead, focus based on your goals:
- Want to be a data analyst? Start with Excel, SQL, dashboards and basic stats
- Aiming for data science? Go deeper into Python, machine learning and experiments
- Interested in ML engineering? Learn deployment, APIs, cloud and system design
Pick a lane, build depth and grow from there.
Quick Checklist for Beginner Data Scientists:
- Think about ethics and privacy in every project
- Understand what the business really needs
- Explain your findings in simple language
- Learn how deployment works, even just the basics
- Write clean, reusable code with good documentation
- Keep learning and stay up to date
- Use automation tools wisely, not blindly
- Practice with messy, real-world datasets
- Ask for feedback often and improve continuously
- Don’t try to learn everything focus on what fits your goals
Have you run into any of these mistakes?
Feel free to share your experience. We'd love to hear how your data science journey is going.
Also read: How to Prepare for a Data Science Interview
Final Thoughts: Think Beyond the Code
Most beginner mistakes in data science aren’t about bad models, they’re about missing the bigger picture.
If you focus only on tools and algorithms, you might miss what really matters: ethical responsibility, business value, clear communication and continuous learning.
Start building those habits now and you’ll stand out from the crowd, while also delivering real impact.
Lorem ipsum dolor sit amet, consectetur adipiscing elit. Suspendisse varius enim in eros elementum tristique. Duis cursus, mi quis viverra.